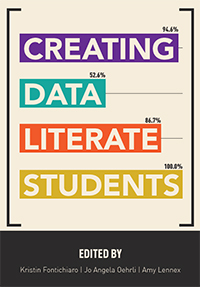
Creating Data Literate Students
Skip other details (including permanent urls, DOI, citation information) :This work is licensed under a Creative Commons Attribution-NonCommercial-NoDerivatives 4.0 International License. Please contact : [email protected] to use this work in a way not covered by the license.
For more information, read Michigan Publishing's access and usage policy.
11 Teaching data contexts: An instructional lens
In textbooks, data often appears next to text that provides context and guidance about how to interpret that data. In the real world, however, disaggregated data often appears disconnected from any text that students might look to for explanation. Bits of data are snagged, aggregated and displayed, often with little context (Weinberger 2007). We encounter them as infographics, unattributed news, data factoids, Google “answers” and even T-shirts (Figure 1). Educators can just accept these “answers” or they can apply an instructional framework of information literacy reasoning (ACRL 2016) to data and treat them as opportunities for inquiry.
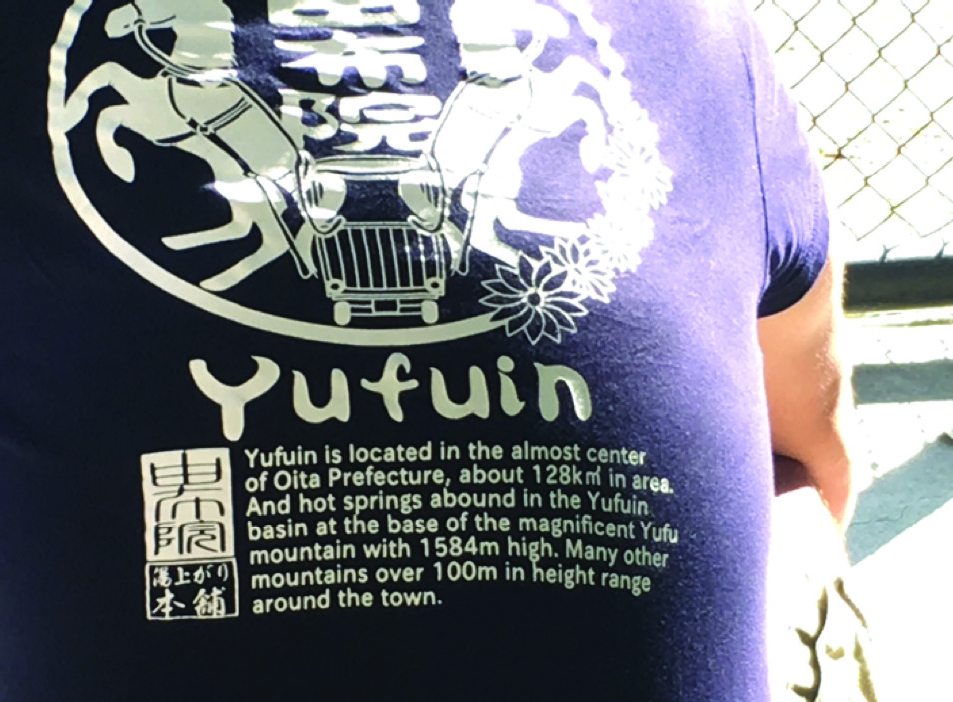
Figure 1: Data presented on a t-shirt at a baseball game. Copyright Debbie Abilock, used with permission.
A statistic doesn’t speak for itself, by itself. As readers, we contextualize statistics to make meaning or evaluate an argument. As authors, we extract and manipulate statistics from data sources to use as evidence within an argument. As the Association of College and Research Librarians’ ACRL Framework for Information Literacy (2016) points out, information is constructed and contextual. Sources reflect the “creators’ expertise and credibility, and are evaluated based on the information need and the context in which the information will be used ... [V]arious communities may recognize different types of authority. It is contextual in that the information need may help to determine the level of authority required.” The same is true of data. As you have seen elsewhere in this book, we must train ourselves to think of numerical data not as truth but as a reflection of the world in which the data was defined, collected, and discussed.
The following scenarios follow a school librarian who is focused on including data literacy in her instruction, specifically framing it within an understanding of data context. By no means are her responses the only possible ones; they depend on the data that’s both available and relevant to the expertise of the students, the goals of the teacher, and our own inclinations and understandings.
Scenario 1: Area: Why context matters
A student needs the area of Alaska for a project he’s doing about the impact of glaciers on global warming. Because he is in the early phases of research, his librarian recommends that he use the open Web to gain easy-to-read, easy-to-access basic information. He uses the search terms [size of Alaska] in an early Google search (note: the brackets are a convention representing the search box but are not characters entered into the search box). Google’s algorithms can now predict the likely kind of information the searcher desires (likely because the word “size” is part of the query) and offer an immediate answer in lieu of a link to a potential answer (see Figure 2).
Alaska must be a pretty big state, he thinks. Another quick search confirms that it’s the largest ... but why are three area numbers listed in the Wikipedia chart: total area (his number), land area, and water area (Figure 3)? He assumed that he’d get a single number answer — a fixed and immutable “area” statistic. He asks the librarian if he should use the total area, which includes water bodies like inland lakes, rivers and even the territorial waters around the land, or the land area which, in the case of Alaska, includes glaciers — that are melting.
This question is important to the student because glaciers are water ... but also solid, like land. The librarian knows that everyday words may imply quantities, counting and measuring but that students may not grasp their significance.
The librarian explains to the student that certain everyday words like area imply a measurement or quantity but, because it is used in various situations, its definition may be ambiguous or imprecise. In this case geologists, geophysicists and other scientists are precise about the area measure they are using (e.g., land or water), so that they can compare the appropriate numbers with others who are measuring glacial recession. Since the whole class will encounter the same ambiguity in area measurements, the librarian decides that this nuance merits greater attention and begins to curate specific information that will help students understand which number to use and why.
An instructor curates to help students develop context or background specific to their needs and the learning goal. Curation is not just linking to a bunch of sources about climate change or glaciers. Google does that. For a librarian to curate for a project means having a conversation with the teacher about the learning goal and the students’ needs, then evaluating and selecting from the “glut” of sources just those which provide the context needed for the students’ investigation. Curation is a targeted instructional strategy librarians can use to build just-in-time background (Abilock n.d.).
Knowing that other schools might also study the effects of climate change on Alaskan glaciers, the librarian taps into social curation tools and searches curated curricular resources built by librarians for use in their academic, special library and school communities (Valenza et al. 2014). The results are abundant, to say the least, so she runs her thinking by the teacher. She proposes limiting this initial curation to specific pages from the U.S. Geological Survey, the major source of U.S. earth science data. Her final list (below) is highly selective and annotated to clarify why each site is relevant and important:
- Definition from the U.S. Geological Survey’s Glaciology Project (http://www.usgs.gov/climate_landuse/clu_rd/glacierstudies/massBalance.asp) This site explains how the area and thickness of glaciers are calculated. The USGS’ Glaciology Project measures how glaciers respond to climate change in order to both predict and prepare for the impact of glacial changes. Read their description of the significance of this glacial mass research to climate change study (http:// www2.usgs.gov/climate_landuse/clu_rd/glacierstudies/ benchmarkGlaciers.asp).
- Visual explanation from Researchers at the Centre for Quaternary Research at Royal Holloway, University of London (http://www.antarcticglaciers.org/modern-glaciers/introduction-glacier-mass-balance/) This site charts explaining glacier mass balance and graphs showing trends of mass balance over time written by a glaciologist as part of her commitment to education.
- Arctic Sea Ice Thickness Maps Data from the Center for Polar Observation and Modeling (CPOM) (http://www.cpom.ucl.ac.uk/csopr/seaice.html) CPOM compares satellite radar signals that bounce off ice vs. water and, together with ice concentration and types data, can produce accurate thickness measurements in near-real time. For additional insight on how satellites can be used to measure glaciers, see http://www.indiaenvironmentportal.org.in/files/file/Arctic%20sea%20ice%20warm%20winter.pdf.
- Glacier Mass Balance Data from the National Snow and Ice Data Center (https://nsidc.org/data/g10002) Historical data from 1945-2003 is available for download. The National Snow and Ice Data Center (NSIDC) manages data and supports research about the cryosphere.
She plans to do a mini-lesson to introduce these sources, then help students find data in CPOM and NSIDC related to their selected glaciers. The teacher, who is much more interested in getting into the action-research project, will use the U.S. Climate Resilience Toolkit framework (https://toolkit.climate.gov), a source that the librarian found, to help the class define the problems, develop solutions based on what they’ve learned, and decide on actions they might take.
Wrap-up
In this first scenario, the librarian recognizes that the student believes he is searching for an unambiguous term that stood for a single number. Rather than treat this as one student’s confusion, the librarian reframes the misconception in a way that can help the entire class recognize that scientists collect specifically-defined measurements over time in order to see trends, make predictions and take action. Her just-in-time curated resources focus on the area measurements that students need for their class’ science project. Her annotations explain why these sources are relevant and authoritative, modeling the critical thinking she wants students to use when evaluating other sources for the project. Collaborative planning between the teacher and librarian take into account their respective strengths and goals.
Try this
For state or country reports, ask students to find the area in square miles (or square kilometers) using Google search results, Wolfram Alpha, a government source or other appropriate sources. What reasons might account for differences? (For more guidance, please see https://www2.census.gov/geo/pdfs/reference/GARM/Ch15GARM.pdf.)
Here are examples of results for Alaska:
Area in square miles | Source |
---|---|
570,640.95 | United States Census (http://www.census.gov/quickfacts/table/LND110210/00,02) |
663,300 | Google (https://www.google.com/search?q=area+of+alaska&ie=utf-8&oe=utf-8) |
661,957 | Alaska Government publication (p.8) (http://labor.alaska.gov/research/pop/estimates/pub/popover.pdf) |
665,400 | Wolfram Alpha (https://www.wolframalpha.com/input/?i=area+of+alaska) |
Here are examples of results for Great Britain:
Area in square miles | Source |
---|---|
84,440 | Wolfram Alpha (https://www.wolframalpha.com/input/?i=area+of+Great+Britain) |
88,745 | Google (goo.gl/PFYucQ) |
80,823 (given as 209331.1 sq. km) | United Nations (http://islands.unep.ch/ICJ.htm#943) |
94,525 | Nations Encyclopedia (http://www.nationsencyclopedia.com/economies/Europe/United-Kingdom.html) |
Scenario 2: Population: How data is constructed and contextualized
American history classes are studying shifts in people’s views about immigration. The teachers plan to contrast the colonial immigrants’ attitudes toward the indigenous Native American population with U.S. citizens’ responses to recent immigrant groups. The librarian knows that the teachers want students to infer historical attitudes from primary sources like letters, journals, diaries, and drawings that they’ve used before. She realizes that she has an opportunity to add data as another type of primary source: a census and a survey. She plans to ask students to draw inferences about societal attitudes by comparing them over time.
She reasons that students should learn about the U.S. Census data because it’s the main source of U.S. population data. Besides providing the population counts that are used to determine the number of seats each state has in the House of Representatives, the results are used “for many important but overlooked political, economic, and social decisions that end up affecting our daily lives” (U.S. Census Bureau, “Why it’s important” n.d.).
Although statistics from public opinion polls are reported ubiquitously today, she is less certain about how to find the primary sources of public opinion survey data from which particular statistics are extracted. Many LibGuides from colleges and universities point to the SDA Archive, which uses the General Social Survey (GSS) and the American National Election Study (ANES), two major sources of public opinion research in the United States. However, after trying to search them herself, she decides that they are too complex for high school students. Instead she discovers the beta GSS Explorer (https://gssdataexplorer.norc.org/), which students can easily use to search, extract, analyze, and even visualize statistics from their longitudinal public opinion surveys run since 1972.
At the social studies departmental meeting she proposes that she teach students to use a public opinion survey and the census to trace public attitudes. She’ll use Alaska as a model, reserving the thirteen original colonies and their states for the students’ own research. She quickly shows teachers how to search the U.S. Census to find population figures, starting with American Indians and Alaskan Natives in Alaska’s 2010 census figures (U.S. Census Bureau, “Quick Facts: Alaska,” n.d.). Then, using five slides, she explains how the Census’s evolving definitions of “Indian” mirrors the societal shifts in attitudes toward race and immigrants:
- Slide #1 Definition #1: The 1787 Constitution established the census to allocate the number of representatives from each state and determine how taxes would be divided among the states. Initially Indians weren’t included for either purpose — they were not considered federal or state citizens because they didn’t pay taxes — so they weren’t counted.
- Slide #2 Definition #2: The American Indian wars and large-scale removals exacerbated distinctions between tribal membership vs. citizenship for the U.S. Marshals, the 1860 census counters, because they were told to count Indians — but only those who had “renounced tribal rule.” (See Figure 4.)
- Slide #3 The census takers were instructed to exclude Indians on reservations, those that were roaming unsettled areas and any Indians living in Alaska (Collins 2006). Although people thought that Indians had clear physical characteristics, the census had no instruction on how to identify them, especially those that were of mixed race living within the general population. No surprise that the marshals found it difficult to count everyone.
- Slide #4 Definition #3: The 1890 census instructed that “all Indians” were to be counted — except neither Aleuts nor Eskimos were included as Indians until Alaska became a state (1959).
- Slide #5 Definition #4: When the government began mailing the census to homes (1960), people were asked to self-identify as Indian. However, people of Hispanic origin self-identified as Indian in large numbers in the 2010 census, muddying the definition still further (U.S. Census, “Instructions” n.d.; Decker 2011).
She demonstrates how to use Pew’s language timeline to pinpoint when racial terms were added or changed in census questions (Pew Research Center for Social and Demographic Trends 2015b). Then she suggests that, when historical periods and immigrant groups are discussed in class, teachers refer to that timeline and prompt students to consider why the government would want to count that group, in that way, at that time.
When classes are ready to look at contemporary attitudes, she will devote another library period to having students read a historical analysis of attitudes toward immigrants that researchers derived from opinion-polling archives (DeSilver 2015). During a third library period, she’ll help students create accounts at the GSS Explorer in order to select and visualize statistical data, evaluate survey methods, and discuss their reflection of attitudes. In a fourth library period, she will ask students to contrast a census with a survey as a formative assessment. In particular, they’ll use a Pew Research survey, looking for potential bias or distortions in the types of questions, then compare it with the proposed data collection plan for the next decennial census (Pew Research Center for U.S. Politics and Policy 2016; Cohn 2015).
After both the science and social studies projects are finished, she’ll want to give students a short online quiz in which they compare the kinds of primary sources used in science vs. social science. For now, she’ll use the C3 social studies standards during library class (see Figure 5) to build their specific awareness about the forms of primary source data that various social scientists use to answer questions in their particular field.
Wrap-up
In this second scenario, the librarian recognizes that the history teachers have overlooked data as a type of primary source. She uses the U.S. Census, a comprehensive longitudinal public data aggregation of U.S. demographic and economic information, because of its importance in government policy decisions as well as in the electoral process. Since students often encounter surveys and polls in popular culture, her goal is to have students distinguish between a survey (which samples a representative subset of the population to make estimates about the entire population) and a census (which aims to gather data from every person in a country). In addition to her explicit focus on how changing definitions and question wording impact data collection and interpretation, she embeds this sequence of lessons within her longer-term scope and sequence plans to integrate vertically across grades and to make curricular connections across disciplines.
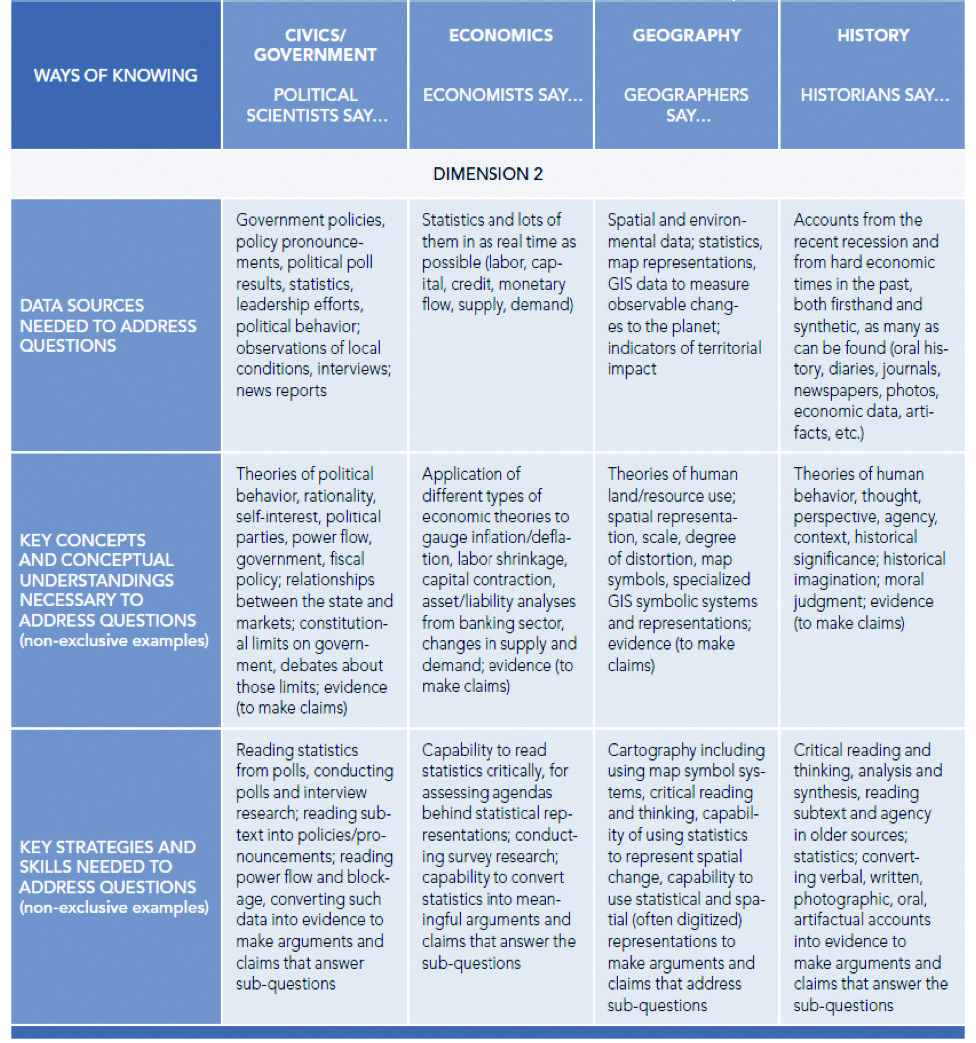
Figure 5: Dimension 2 of the C3 Framework showing the kinds of data needed to address disciplinary research questions and the specific reading strategies needed (National Council for the Social Studies 2013). Citation: National Council for the Social Studies (NCSS), The College, Career, and Civic Life (C3) Framework for Social Studies State Standards: Guidance for Enhancing the Rigor of K-12 Civics, Economics, Geography, and History (Silver Spring, MD: NCSS, 2013).
Try this
While some countries collect data about race, ethnicity or religion, it illegal in others to ask questions about these topics in a census (INSEE 2015). Look for opportunities in language classes or global studies where students could look at the social and cultural contexts of census data in other countries.
Scenario 3: Pandemics: The emotional context
Students’ fears about catching Ebola or the Zika virus have spiraled as reports about deaths and birth defects flood the news. The combination of unusual symptoms, impact on infants, and limited prevention information, along with emotionally-charged graphic descriptions of transmission and high death rates, are a sure recipe that an “availability bias” that will color how students respond to data reported in the news. Availability bias is a psychological term referring to how the mind can give greater weight to the newest or most familiar information. When data is presented as odd (unique or unusual) and memories are recent and filled with anxiety, one is likely to overestimate the likelihood of something bad happening.
A teacher and the librarian decide to do a health unit to help students manage these gut-level responses. They want students to slow down their thinking, so they decide to add moments of “friction” to the lesson (Seroff, Bergson-Michelson, and Abilock 2015). By doing so, they hope students will learn to step back from knee-jerk emotions to perform a more dispassionate evaluation of news about disease outbreaks and quantify their risks analytically. There is particular urgency in learning this because dissemination of information about epidemics in social media has preempted the traditional role of health officials who normally issue warnings on authoritative websites with full explanations of symptoms, risks and prevention plans (see, for example, the World Health Organization (WHO) and its Disease Outbreak News alerts posted at http://www.who.int/csr/don/en/). Indeed, a recent study found that tweets, not official WHO Disease Outbreak News (DONs), broke the Ebola story to over 60 million people over a three-day period (Odlum and Yoon 2015).
The teacher and the librarian acknowledge that they, probably like their students, are unclear about the differences between commonly-used terms like epidemics and pandemics, so they look for background information from the Centers for Disease Control and Prevention (CDC), the major source of health data about Americans:
The amount of a particular disease that is usually present in a community is referred to as the baseline or endemic level of the disease. This level is not necessarily the desired level, which may in fact be zero, but rather is the observed level. In the absence of intervention and assuming that the level is not high enough to deplete the pool of susceptible persons, the disease may continue to occur at this level indefinitely. Thus, the baseline level is often regarded as the expected level of the disease (CDC 2012).
Occasionally, the amount of disease in a community rises above the expected level. Epidemic refers to an increase, often sudden, in the number of cases of a disease above what is normally expected in that population in that area. Outbreak carries the same definition of epidemic, but is often used for a more limited geographic area. Cluster refers to an aggregation of cases grouped in place and time that are suspected to be greater than the number expected, even though the expected number may not be known. Pandemic refers to an epidemic that has spread over several countries or continents, usually affecting many people (CDC 2012a).
The teachers decide to focus on infectious diseases like Zika and Ebola, rather than including non-contagious epidemics like diabetes and obesity. Their first thought is to prompt students with headlines about epidemics that include or imply statistics, but locating a sufficient number proves to be too laborious. Instead they locate a fear-based infographic that received quite a bit of traction when it was released (https:// www.good.is/infographics/infographic-the-deadliest-disease-outbreaks-in-history).
Students pick a disease from the infographic — one that either worries or interests them — and then team up by their chosen disease. Groups will deconstruct the data given in the infographic and compare it to information about the disease from other sources. Knowing that students’ free-text searches will return all sorts of random data bits, the teacher and librarian decide to limit students’ searches to two sources of reasonably comparable data about pandemics:
- The Centers for Disease Control and Prevention contains a rich range of data with a focus on Americans, ranging from simple statistics in FastStats (http://www.cdc.gov/nchs/fastats/) to raw datasets on diseases and conditions (http://www.cdc.gov/DiseasesConditions).
- The World Health Organization, the major international source of disease data, publishes Global Health Observatory by country (http://www.who.int/gho/en) and by topic (http://www.who.int/topics/en).[4]
To be sure that all groups collect similar information, the instructors create a spreadsheet-like matrix to contain the data found by each group (http:// noodle.to/pandemic). To maximize their efficiency while researching and recording data, the teachers add the following links to the matrix:
- A link to the infographic
- A source for historical census data
- Links to the CDC and WHO information about infectious diseases
- Definitions of key terms students will encounter: endemic, epidemic and pandemic
Since they want students to also evaluate the visual display of the data in the infographic, they include a column for a statistic called the Case Fatality Rate (CFR), which quantifies the deaths among cases.
In data literacy, it is essential that students understand how to select comparable numbers. For example, the Zika outbreak in Brazil that began in April 2015 ought to be matched with data from the same period (2015-2016), some of which is likely to be an estimate. They show students a matrix of Brazil’s population data collected from various sources (goo.gl/0p4Se1) and remind them to avoid a “precision bias,” a cognitive predisposition to assume that the most precise number they find is de facto the most accurate.
During a class discussion, they consider other evaluation criteria for Brazilian population statistics. Should they use http://Worldometers.info, which seems to update in real time but is clearly an estimate? Should they use some average of the population data from the same year(s)? Perhaps they should use the Brazilian Census figures because they’re “official” government numbers?
Ultimately, they decide to use data from The World Bank because it standardizes Brazil’s aggregated data from global, national and regional sources so that it’s comparable with other countries’ data. The online interface allows them to visualize the data on maps or in graphs, view it in tables or download it as datasets. Students also find the Bank’s country office contacts in Brazil and Washington D.C. and the names of a specific team focused on combatting Zika in Latin American that they might email with questions. The availability of expert help, the comparable numbers from identifiable sources, and the prospect of experimenting with varied visualizations outweigh the students’ initial preference for data sites that offer easier access and simpler results.
As students start to compare U.S.-focused information with global data, they realize that there are differences in the case fatality ratio (CFR), which is the risk of death expressed as a percent based on the proportion of people who die from a disease outbreak out of those who are infected. CFR will differ based on regional or local conditions and health care systems. For example, while measles is currently under control (endemic — and therefore not much of a worry) in countries like the U.S., it is the leading cause of death among children in India, Nigeria and Pakistan (CDC 2015).
Once the teams complete the matrix, each group presents their findings to the class, comparing them with what was displayed in the infographic. As they present, the teacher makes connections to other measures of morbidity, such as the number of Brazilians who die from other causes like heart disease and cancer. This helps students contextualize the scope of the outbreak (CDC 2012a).
Next the teachers want the class to practice using data selectively from the matrix as evidence in an argument. To help students appreciate how the same data can be used to support very different conclusions, they show a New York Times interactive graphic that models using the same job numbers for different arguments (http://www. nytimes.com/interactive/2012/10/05/business/economy/one-report-diverging-perspectives.html). The class discusses how drawing different conclusions from the same data can be done with complete honesty; it’s not always a signal of intentional manipulation.
The librarian reinforces this idea by explaining one experiment reported in Nature in which 29 scientific teams looked at the same information about soccer games and answered the same question: “Are dark-skinned players more likely to be given red cards than light-skinned ones?” The scientists came to widely disparate conclusions; some saw no difference between light- and dark-skinned players while others saw a strong trend toward giving more red cards to dark-skinned players. The study concludes that each team’s inferences were contextualized by their expertise and background:
Teams approached the data with a wide array of analytical techniques, and obtained highly varied results. Next, we organized rounds of peer feedback, technique refinement and joint discussion to see whether the initial variety could be channeled into a joint conclusion. We found that the overall group consensus was much more tentative than would be expected from a single-team analysis (Silberzahn and Uhlmann 2015).
As students become more comfortable with data literacy skills, they approach ambiguities in exercises like these with greater confidence and are open to understanding that data is neither infallible nor arbitrary — human interpretation plays a key role.
Teachers assign the “Infographic Design Matrix” to help students identify their audience (Abilock and Williams 2014). As a final task, each student will select some statistics from the matrix to support a unique visual argument — to display either on a PowerPoint slide or, if they have time, as an infographic — using some of the statistics they have collected. Teachers caution students to resist “anchoring,” another cognitive bias in which students accept the first piece of evidence as “truth” and measure all other information against that first data bit. The teacher and librarian hope that this selection process reinforces students’ understanding that data is being used as evidence rather than as immutable facts.
Wrap-up
In this scenario, the librarian and teacher decide to look at a visual display of decontextualized data — what we commonly call “data in the wild.” Since infographics are so prevalent online, they want students to both deconstruct and, at least partially, to construct their own visual display of data using a typical genre. They explain to students that, to make comparisons easier, they are controlling both the sources of data and the format in which statistics are collected. They offer students a limited choice so that students will be able to compare and discuss comparable results. Initially students work in groups but, to ensure accountability, they eventually choose data that is relevant to a specific audience in order to craft a compelling argument. Throughout the project, the teacher and librarian continually refer to ways in which data is constructed and contextualized — by cognitive biases, content creators, a purpose and an audience.
Try this
Explore data context. Context can mean both the genre in which numbers are found (e.g., chart, spreadsheet, infographic, or text) as well as the content described by the numbers. Expose students to spreadsheets of data that compare political, social or economic aspects of countries. When seemingly comparable numbers appear in the same spreadsheet, students make superficial comparisons simply because the numbers seem related. In UNICEF’s single spreadsheet (http://data.unicef.org/topic/child-protection/child-labour/, in “Access the Data” section), Afghanistan and Chile have similar data on percentages of child labor by country. However, the circumstances within each country, as well as the absolute numbers of children, are vastly different. In Afghanistan, most children work in agriculture, in their homes, in forced hazardous brick production or in illicit activities. In Chile, most are in retail businesses or commercial sexual exploitation. In Chile, a prosperous country, there is a significant government push to eliminate the worst forms of child labor, an initiative that Afghanistan, a poor nation, would find much more difficult to implement.
Notes
4. Both the National Center for Health Statistics (NCHS) and the World
Health Organization (WHO) are developing easy-to-use online tools that
allow users to examine vital statistics data interactively and create
their own tables within the tool, as well as export data for use in
other formats. Since these major sources for public health data are
likely to be valuable for future research projects (e.g., maternal and
child health, nutrition, dental care, substance abuse, noninfectious
diseases), school librarians might want to explore them now and follow
their evolution.
Strategies for teaching context in the wild: Find a problem, build a rule
Both the explicit guidelines that can help novices learn to vet the credibility of new content and the tacit “rules of thumb” that they subconsciously use to evaluate familiar content are part of the “context” we bring to data literacy. Typically, each of us makes unconscious decisions in many, daily situations where we have to make a choice (Gigerenzer 2007). We use mental shortcuts, called heuristics, to speed decision-making. These unarticulated “rules” — which may begin with formally stated recommendations but then transition into tacit, intuitive behaviors — allow us to function efficiently without stopping to think through each choice we make, each action we take, and each detail of a problem we encounter.
These shortcuts are generally good enough. We happily perk along unconsciously using these rules — until they don’t work. When we recognize that we are stuck, we will bring the rule into consciousness (metacognition) and consider revising it. Good instructional design aims to bring these rules to light by putting challenges in front of students so that they reexamine their assumptions, learn from their errors and revise simplistic algorithms.
The general problem with relying on unconscious rules of thumb is that they reinforce cognitive biases. And, while a set of explicit data literacy “rules” may provide guidance for beginners, such lists are not productive in the long run. Students will change rule-based behavior only when cognitive dissonance provokes a shift in their thinking. As Kuhn (2000) asserts, “Strategy training may appear successful, but if nothing has been done to influence the metalevel, the new behavior will quickly disappear once the instructional context is withdrawn and individuals resume metalevel management of their own behavior.”
Another possible teaching strategy is to use discussion and reflection to uncover the useful tacit knowledge within rules of thumb (Polanyi and Sen 2009; André et al. 2002). Initially we can ask students to become aware of their unconscious rules by completing the following sentence:
“When I see...then I do...”
so that they identify and then describe a specific and conditional decision strategy that they employ in a particular situation. In the process of explaining a rule, students may verbalize strategies that their peers have not considered. Or they may be able to convert a vague rule of thumb into a just-in-time checklist, which is what Gawande argues is necessary for critical decision-making in highly charged situations like an operating room or the cockpit of a falling plane (Gawande 2010). One opening instructional move, then, is to have students develop their own checklists targeted to places where it’s essential to make critical decisions about data evaluation or data visualization. For example, we may teach a novice to look where zero falls on the y-axis of a graph. Over time, however, a student may revise that to a more nuanced checklist:
- Don’t assume that the y-axis begins at zero.
- Look for labels on the y-axis.
- Look at the increments on the y-axis to help you know if a change is significant or not.
Of course, experts also have rules of thumb that we can learn from; these are valuable procedures and processes that emerge from their years of experience within a discipline or field. One set of processes specifically related to data literacy is described by a professor of sociology and criminal justice as “statistical benchmarks.” As mentioned throughout this text, these are validated statistics (such as the size of the U.S. population) that can help us judge whether new population statistics we encounter are significant (Best 2013). While we may not have the disciplinary expertise to provide students with benchmark strategies for every topic, we can model a process that involves noticing a problem with odd data, unearthing our tacit assumptions, faulty procedures and unconscious misconceptions and then developing more accurate strategies to evaluate data in the wild. Let’s explore what this might look like in the following four short scenarios.
Example 1: Evaluating data in the context of a visualized benchmark
Scenario: The Internet’s Own Boy
The Internet’s Own Boy is a documentary film about programming prodigy and open-access activist Aaron Swartz. In the film, public domain advocate Carl Malamud agrees to work with Swartz to download and provide free access to what are, in fact, public records. Indeed, the Public Access to Court Electronic Records (PACER) database makes inordinate profits on what should be freely accessible court records (E-Government Act of 2002; Internet’s Own Boy 2014 31:33 min). As a result of their activism, PACER agrees to provide free access to 17 libraries across the country. Malamud exclaims: “One library for every 22,000 square miles!” Does this make any sense, even in a quick mental check that requires only a few seconds?
Finding a relevant statistical benchmark
When I saw this film, I referenced a quick benchmark from my toolkit: the fact that the 48 contiguous states make a very rough rectangle about 3,000 miles from east to west and perhaps about 1,000 miles from north to south: an area of about 3 million square miles. 3 million square miles divided by 17 locations? It’s instantly clear that there are far too few locations for the majority of Americans to reach easily, even without figuring in Alaska and Hawaii. Another way I could have approached this would have been to use the benchmark that we have 50 states. 50 states divided by 17 locations means around one location for every three states: again, not very accessible for most Americans. A third way to approach this would be to have the actual total area of the United States in mind as a statistical benchmark. According to the U.S. Census, the total land and water mass of the United States (including Hawaii and Alaska) is 3,805,927 square miles (U.S. Census 2012). Again, it’s very quick to see that 3.8 million divided by 17 is very poor coverage.
Reasoning using the statistical benchmark
So the average area covered by each of those 17 libraries would be about 3.8 million square miles divided by 17 libraries, which is a little over 220,000 square miles. It’s beginning to look like that
22,000 figure is off by a full order of magnitude and might be attributable to a decimal point error.
Example 2: Evaluating data in the context of a common misconception
Scenario: The Martian
The film The Martian (2014) tells the story of a manned mission to Mars that goes awry because the crew leaves one man for dead. NASA realizes he’s still alive and pulls out all the stops to bring him home. Under tremendous pressure to launch a rescue ship and worried about the astronaut’s mental as well as physical health, Vince Kapoor (Chiwetel Ejiofor) says, “He’s 50 million miles away from home, he thinks he’s totally alone, he thinks we gave up on him — I mean, what does that do to a man, psychologically? What the hell is he thinking right now?” (2015, 34:10 min). Is that 50 million mile distance from Earth to Mars credible within the context of the film’s story?
Uncovering a data misconception with students
From their first picture book about the solar system to endless examples in media, students have been exposed to distorted images of the planets, their orbits and distances. Help students develop a better idea of the actual distances using a video showing a scale model of the solar system on a dry lakebed in Nevada (https://vimeo.com/139407849).
Reasoning through a data misconception with students
Many students have already learned that Earth’s average distance from the sun is about 93 million miles, and a quick Web check confirms that Mars’ average distance from the sun is about 142 million miles. So it’s obvious that the screenwriters probably just subtracted one figure from the other to arrive at 50 million miles. In fact, the two planets orbit the sun at different speeds and come that close to each other only on rare occasions when their positions in their orbits lined up on the same side of the sun. They do not remain the same distance apart throughout their orbits. Indeed, the Mars Mission Director’s exclamation would have been more dramatic (and more credible), if he had relegated the stranded astronaut to a position twice as far from home!
Example 3: Answering a data question by making an analogy to a known data context
Scenario: Historical data on the Black Death
Recently I asked an epidemiologist how we could teach students to figure the case fatality ratio — remember that’s the number who die out of the number who get infected — for the Black Death. She acknowledged that historical population numbers, infection rates, and death rates are very rough estimates.
Finding a known context and using it as an estimated proxy
She shared a strategy that public health workers use to assess outbreaks of diseases that have a historical trail. Yersinia pestis, the bacterium responsible for the Black Death, the Plague of Justinian, and the Third Plague, continues to cause plagues in Africa and Asia today (CDC 2015). Therefore, to estimate a historical disease impact like the Black Death’s case fatality ratio she uses the modern case fatality ratio assuming no treatment, since there were no antibiotics during the 14th century.
Reasoning through the data analogy with students
By applying current-day CFR estimates for plagues to Black Death, we can guess that the CFR ranged from about 50% (for the bubonic form) to almost 100% (for the pneumonic form). Of course, CFRs are a moving target. In both historical and modern times, the bubonic plague affects the old and infirm in the first wave, but death rates drop significantly as immunity builds and the weak are wiped out — and so the CFR declines.
Scenario: Unemployment figures
“We have 93 million people out of work. They look for jobs, they give up, and all of a sudden, statistically, they’re considered employed” (Jacobson 2015). This seems like an enormous and very serious problem — and it’s repeated often, more recently upped to 94 million. People assume that “out of work” means “unemployed,” that is, 93 million people want to work and are looking for a job but can’t find one.
Finding a context
Two statistical benchmarks for students to remember are the current population of the U.S. (about 325 million) and China (about 1.3 billion). If we go to the Bureau of Labor Statistics (BLS), a good source of government information about jobs, we find that the U.S. unemployment rate is currently about 5% (BLS 2016). If 93 million people represents 5% of the U.S. population, the total U.S. population would have to be almost 2 billion people. That doesn’t make sense — even the population of China is only somewhere over one billion!
Reasoning through the statistical claim with students
The BLS issues a monthly press release on the number of unemployed people — currently about 8 million (BLS 2016). In fact, 93 million people are not “out of work” (i.e., unemployed) but rather they are “out of the workforce.” Most of this number consists of people of working age who aren’t looking for jobs — students, disabled people, housewives/househusbands, early retirees — anyone who could theoretically work. Ask students to think about whether the recurring choice of the phrase “out of work” or “out of the labor force” in these claims involves ignorance or intent to deceive.
By gathering examples like these from popular culture, politics, and the media, we can support students as they recognize and wrestle with real-world data challenges.
Conclusion
Throughout this chapter we have modeled teaching strategies to scaffold students’ growing understanding and ability to evaluate data in the wild. By contextual framing, we can address students’ grab-and-go approach to data and create moments of friction (Abilock 2016) at which point they are intrigued enough to reassess their assumptions about numbers as indisputable and fixed. Ambiguity drives inquiry. Investigations of data context result in data insights. As educators, we can choose when our students are ready to tackle this ambiguity and, by doing so, achieve higher levels of data comprehension.
Resources
- Abilock, Debbie. n.d. “Curriculum Curation.” OER Commons. Accessed April 24, 2017. https://www.oercommons.org/courseware/module/11007 .
- _______. 2016. “How Can I Teach Students to Think of Numbers as Evidence Rather than Answers?” School Library Connection, March, 40-41.
- Abilock, Debbie, and Connie Williams. 2014. “Recipe for an Infographic.” Knowledge Quest 43(2), November/December, 46-55. Accessed April 19, 2017. http://files.eric.ed.gov/fulltext/EJ1045949.pdf.
- André, Malin, Lars Borgquist, Mats Foldevi, and Sigvard Mölstad. 2002. “Asking for ‘Rules of Thumb’: A Way to Discover Tacit Knowledge in General Practice.” Family Practice 19(6), December, 617-22. doi:10.1093/fampra/19.6.617 .
- Association of College and Research Libraries. 2016. “Framework for Information Literacy for Higher Education.” ALA. Accessed April 19, 2017. http://www.ala.org/acrl/standards/ilframework.
- Best, Joel. 2013. Stat-spotting: A Field Guide to Identifying Dubious Data. Updated and expanded ed. Berkeley: University of California Press.
- Bostok, Mike, Amanda Cox, and Kevin Quelay. 2012. “One Report, Diverging Perspectives.” New York Times, October 5. http://www.nytimes.com/interactive/2012/10/05/business/economy/one-report-diverging-perspectives.html .
- Bureau of Labor Statistics. 2016. “The Employment Situation - April 2016.” News release, May 6. Accessed April 19, 2017. http://www.bls.gov/news.release/pdf/empsit.pdf.
- Centers for Disease Control and Prevention (CDC). 2012a. “Section 11: Epidemic Disease Occurrence.” Accessed April 24, 2017. http://www.cdc.gov/ophss/csels/dsepd/ss1978/lesson1/section11.html.
- _______. 2012b. “Lesson 3: Measures of Risk.” Accessed April 24, 2017. http://www.cdc.gov/ophss/csels/dsepd/ss1978/lesson3/section2.html .
- _______. n.d. “Moving Faster than Measles and Rubella.” Infographic. Accessed April 19, 2017. http://www.cdc.gov/globalhealth/immunization/infographic/measles.htm .
- _______. 2015. “Plague.” Accessed April 24, 2017. http://www.cdc.gov/plague/.
- Cohn, D’Vera. 2015 .”Census Considers New Approach to Asking about Race – by Not Using the Term at All.” FactTank (blog), Pew Research Center. Accessed April 19, 2017. http://www.pewresearch.org/fact-tank/2015/06/18/census-considers-new-approach-to-asking-about-race-by-not-using-the-term-at-all/ .
- Collins, James P. 2006. “Native Americans in the Census, 1860–1890.” Prologue Magazine, 38(2), Summer. Accessed April 19, 2017. http://www.archives.gov/publications/prologue/2006/summer/indian-census.html .
- Column Five. 2011. “Outbreak: The Deadliest Pandemics in History.” Infographic. GOOD. Accessed April 19, 2017. https://www.good.is/infographics/infographic-the-deadliest-disease-outbreaks-in-history#open .
- Decker, Geoffrey. 2011. “Hispanics Identifying Themselves as Indians.” New York Times, July 3. Accessed April 19, 2017. http://nyti.ms/18KBMlo.
- DeSilver, Drew. 2015. “U.S. Public Seldom Has Welcomed Refugees into Country.” FactTank (blog). Pew Research Center, November 19. http://www.pewresearch.org/fact-tank/2015/11/19/u-s-public-seldom-has-welcomed-refugees-into-country/ .
- E-Government Act of 2002. 107–347 107th Congress. Accessed April 24, 2017. https://www.gpo.gov/fdsys/pkg/PLAW-107publ347/pdf/PLAW-107publ347.pdf .
- Gawande, Atul. 2010. The Checklist Manifesto: How to Get Things Right. New York: Metropolitan Books.
- Gigerenzer, Gerd. 2007. Gut Feelings: The Intelligence of the Unconscious. New York: Viking.
- Internet’s Own Boy, The: The Story of Aaron Swartz. Directed by Brian Knappenberger. 2014. Beverly Hills, CA: Participant Media, 2015. DVD.
- Jacobson, Louis. 2015. “Donald Trump Says U.S. Has 93 Million People ‘Out of Work,’ but That’s Way Too High.” Politifact, August 31. Accessed April 19, 2017. http://www.politifact.com/truth-o-meter/statements/2015/aug/31/donald-trump/donald-trump-says-us-has-93-milion-people-out-work/ .
- Kuhn, Deanna. 2000. “Does Memory Development Belong on an Endangered Topic List?” Child Development 71(1), January/February, 21-25. doi:10.1111/1467-8624.00114.
- The Martian. 2015. Directed by Ridley Scott. 2015. Beverly Hills, CA: 20th Century Fox, 2016. DVD.
- Murphy, Nora. 2016. “How to Develop Strong Source Literacy: Practice!” Voices from The Hill (blog). January 1. Accessed April 19, 2017. http://blog.fsha.org/develop-source-literacy/ .
- National Council for the Social Studies (NCSS). 2013. The College, Career, and Civic Life (C3) Framework for Social Studies State Standards: Guidance for Enhancing the Rigor of K-12 Civics, Economics, Geography, and History. Silver Springs, MD: NCSS,. http://www.socialstudies.org/system/files/c3/C3-Framework-for-Social-Studies.pdf .
- National Institute for Statistics and Economic Studies (INSEE). 2016. “Ethnic-based Statistics.” INSEE, September 16. Accessed April 19, 2017. https://www.insee.fr/en/information/2388586.
- Odlum, Michelle, and Sunmoo Yoon. 2015. “What Can We Learn about the Ebola Outbreak from Tweets?” American Journal of Infection Control 43(6), June 1, 563-71. http://dx.doi.org/10.1016/j.ajic.2015.02.023.
- Pew Research Center for U.S. Politics and Policy. 2016. “Campaign Exposes Fissures Over Issues, Values and How Life Has Changed in the U.S.” Pew Research Center. Accessed April 19, 2017. http://www.people-press.org/2016/03/31/campaign-exposes-fissures-over-issues-values-and-how-life-has-changed-in-the-u-s/ .
- Pew Research Center for Social and Demographic Trends. 2015a. “Chapter 7: The Many Dimensions of Hispanic Racial Identity.” In Multiracial in America: Proud, Diverse and Growing in Numbers, 98-109. Ed. Kim Parker et al. Washington, DC: Pew Research Center. Accessed April 17, 2017. http://www.pewsocialtrends.org/2015/06/11/multiracial-in-america/.
- Pew Research Center for Social and Demographic Trends. 2015b. “What Census Calls Us: A Historical Timeline.” Pew Research Center, June 10. Accessed April 22, 2017. http://www.pewsocialtrends.org/interactives/multiracial-timeline/.
- Polanyi, Michael, and Amartya Sen. 2009. The Tacit Dimension. Chicago: University of Chicago Press.
- Seroff, Jole, Tasha Bergson-Michelson, and Debbie Abilock. 2015. “Friction: Teaching Slow Thinking and Intentionality in Research.” NoodleTools, Accessed November 15, 2015. https://www.noodletools.com/debbie/literacies/information/friction.pdf .
- Silberzahn, Raphael, and Eric H. Uhlmann. 2015. “Crowdsourced Research: Many Hands Make Tight Work.” Nature 526(7572), October 7, 189-91. doi:10.1038/526189a.
- United States Bureau of the Census. 1994. “Chapter 15 Area Measurement/Water Classification.” In Geographic Areas Reference Manual, 15-1-15-11. Washington, DC: Department of Commerce, 1994. Accessed April 24, 2017. https://www2.census.gov/geo/pdfs/reference/GARM/Ch15GARM.pdf.
- United States Census Bureau. n.d. “Census Instructions.” https://www.census.gov/history/www/through_the_decades/census_instructions/ .
- . n.d. “1860 Instructions.” Accessed April 24, 2017. https://www.census.gov/history/www/through_the_decades/census_instructions/1860_instructions.html .
- _______. n.d. “Quick Facts: Alaska.” Accessed April 24, 2017. https://www.census.gov/quickfacts/table/PST045216/02.
- _______. 2012. “State Area Measurements and Internal Point Coordinates.” Accessed April 24, 2017. https://www.census.gov/geo/reference/state-area.html.
- _______. n.d. “Why It’s Important.” Accessed April 24, 2017. http://www.census.gov/2010census/about/why-important.php.
- Valenza, Joyce Kasman, Brenda L. Boyer, and Della Curtis. 2014. Library Technology Reports. 50(7), October. Chicago, IL: ALA TechSource. Accessed April 24, 2017. https://journals.ala.org/index.php/ltr/issue/view/200.
- Weinberger, David. 2007. Everything Is Miscellaneous: The Power of the New Digital Disorder. New York: Times Books.
- World Health Organization (WHO). 2017. “Zika Virus and Complications: Questions and Answers.” Accessed April 24, 2017. http://www.who.int/features/qa/zika/en/.