Factors That Influence the Agreement in Energy Expenditure Assessment in Pediatric Intensive Care Patients
Skip other details (including permanent urls, DOI, citation information)
:
This work is licensed under a Creative Commons Attribution-NonCommercial-ShareAlike 3.0 United States License. Please contact : [email protected] to use this work in a way not covered by the license.
For more information, read Michigan Publishing's access and usage policy.
Abstract
Introduction:
The primary goal of our study was to examine the agreement between the resting energy expenditure (REE) obtained by indirect calorimetry and the initial estimated energy expenditure (EEE) obtained by a nutritionist’s assessment. The secondary goal of our study was to examine factors that might contribute to the observed relationship between REE and EEE.
Methods:
This was a retrospective, observational study conducted at an academic medical center. One hundred eight patients admitted to the pediatric intensive care unit were included in the primary analysis. Analysis of the 108 patients involved a comparison of energy expenditure estimates obtained by indirect calorimetry and nutritionist assessment. Thirty–four of those patients were used in a subset analysis to identify covariates that may affect the observed difference.
Results:
Our primary analysis demonstrated a statistically significant difference between REE and EEE (median difference = 259 kcal/day; IQR = 114–458 kcal/day, P = 0.00). Our secondary analysis identified three factors that were independently associated with this observed difference: the patient’s age, heart rate, and tidal volume setting on the mechanical ventilator. Fifty–seven percent of the observed variability in the difference between REE and EEE was shown to be associated with those three covariates (P < 0.05, R2 = 0.565).
Conclusion:
Because meeting the nutritional needs of pediatric intensive care unit (PICU) patients has been recognized as an important adjunct to improve outcomes, clinicians should focus on certain aspects of the patient’s hemodynamic stability, the patient’s ventilator settings, and the age of the patient whenever a comparison is made between indirect calorimetry and standardized equations for estimating energy expenditure.
Introduction
Meeting the nutritional needs of pediatric intensive care patients has long been recognized as an important adjunct to minimize or prevent complications and improve outcomes.[1]–[7] When energy expenditure estimates do not accurately approximate energy requirements, the patient may be at risk for overfeeding, which can lead to a myriad of complications, including impaired glucose homeostasis, lipid disorders, hepatic steatosis, and increased risk for infection.[8]
The ability to assess energy expenditure can be difficult, especially in pediatric intensive care patients who require mechanical ventilatory support.[4],[9]–[16] In such settings, nutritionists use a combination of set equations often involving stress and growth factors to indirectly determine a patient’s estimated energy expenditure (EEE). Indirect calorimetry (IC) can also be used to provide a real–time look at patients’ metabolic activity to identify the resting energy expenditure (REE). A patient’s nutritional regimen is frequently designed according to the values obtained (EEE and REE); however, these values often differ.[16]–[18]
The primary goal of our study was to examine the agreement between the REE obtained by IC and the initial EEE obtained by the nutritional assessment. The secondary goal of our study was to examine factors that might contribute to the observed relationship between REE and EEE.
Methods
Research Subjects
During the period between April 2011 and February 2014, a total of 171 patients admitted to the pediatric intensive care unit (PICU) at C. S. Mott Children’s Hospital and Von Voigtlander Women’s Hospital were assessed by a nutritionist and tested using an indirect calorimeter, the Vmax Encore System (CareFusion Corp, San Diego, California).[19] Of these 171 patients, 108 were retained for our analysis, following the exclusion criteria displayed in Figure 1. Patients were selected for this study solely based on their requirement for PICU support, regardless of their age, and the request for IC. This study was reviewed and approved by the University of Michigan Institutional Review Board for human subjects and deemed to meet all appropriate standards.
Protocol
A clinical nutritionist determined an estimate of each patient’s energy expenditure using common equations as follows: World Health Organization = 31 (29%), dietary reference intakes = 21 (19%), Schofield = 7 (6%), recommended dietary allowances = 5 (5%), Mifflin = 2 (2%), basal energy expenditure = 2 (2%), Penn State = 1 (1%), or not specified = 40 (37%).[20]–[23] The EEE value obtained by these equations was often multiplied by a factor ranging from 0.6 to 1.45 at the discretion of the nutritionist to account for stress and growth factors. Indirect calorimetry was performed at the request of the attending physician following the nutritionist’s estimate of the patient’s energy expenditure. If the physician sought multiple IC tests—which is not uncommon, seeing that REE likely varies from day to day and as a patient’s condition improves or declines—only the value from the initial test was used in our study. The original value was chosen because it most closely reflected the patient’s condition upon entry to the PICU.
Each patient’s test session included calibration of the Vmax Encore per manufacturer guidelines and subsequent measurement for a minimum period of 15 minutes to obtain “steady state” results.[24] The first 5 minutes were discarded to allow for patient stabilization; a 10–minute average following stabilization was used to calculate the REE. The results obtained representing “steady state” were extrapolated in order to predict energy expenditure over a period of 24 hours; this methodology was validated in previous studies.[25]–[26] The respiratory therapy staff, all of whom were trained on the operation and calibration of the IC cart, attempted to perform the test while the patient was not being stimulated nor experiencing changes in mechanical ventilation approximately 45 minutes prior to and during testing. The data for the REE and respiratory quotient were collected from the Vmax Encore at the completion of the test. Information regarding the patient’s mechanical ventilator settings and vital signs were available and recorded at the time of study.
Statistical Analysis
Descriptive statistics were used to identify the distribution of the data in our study population. The median and interquartile range (IQR) were used for continuous variables, and frequencies were used for dichotomous variables, for both our total and subset analysis. Correlation and linear regression analysis were performed on both the aggregate and subset populations as identified in the results.
Taking into account that the lag time between the EEE and REE may be a confounding factor, we limited our subset study population to only include patients with a lag time equal to 1 calendar day or fewer between the time of the nutritional assessment and IC test date. We chose to use a 24–hour cutoff because patients in an intensive care unit are quite often unstable and are experiencing a constantly changing environment, and if we were to compare the nutritionist’s estimate with an IC value greater than 24 hours apart, the differences in energy expenditure shown may be related to the variability in the patient’s status, such as weaning off the ventilator, starting antibiotics, or changes in sedation level rather than intrinsic differences between energy expenditure as measured by IC and that measured by a clinician. For this reason, our subset cohort, consisting of 34 patients, was used to identify factors or conditions that may influence the difference between nutritionist–based estimates and those obtained from IC. The variables examined included age, weight, body mass index (BMI), severity scores (PRISM III), vital signs (heart rate, temperature, systolic blood pressure, diastolic blood pressure), and mechanical ventilator settings (FiO2, tidal volume, frequency, positive end–expiratory pressure [PEEP]). A stepwise forward linear regression was conducted to identify covariates that were independently associated with the difference between REE and EEE (REE − EEE).
A P value of ≤ 0.05 was selected a priori as indicative of statistical significance in our correlation and regression models. Graphical representation of the data was performed using Excel 2011 (Microsoft, Redmond, Washington), and statistical analysis was conducted using commercially available software (SPSS Version 21.0; SPSS, Inc, an IBM Company, Chicago, Illinois).
Results
Figure 1 identifies the inclusion and exclusion of subjects for this study. An initial population of N = 171 was reduced to N = 108 for reasons outlined in the diagram. A subset of the aggregate population was used for additional analysis to identify covariates independently associated with the observed difference between REE and EEE. Figure 2 demonstrates the Bland Altman plot of the energy expenditure difference (REE − EEE) from the energy expenditure average for all the matched pairs (N = 108). One standard deviation from the mean was +/–362 kcal/day, and 2 standard deviations from the mean was +/–723 kcal/day. The 2 horizontal lines on the graph represent +/–2 standard deviations.
Based on the data collected for our observational study, the lag time between the estimated assessment and the measured assessment was noted to be as great as 7 days. The relative percentage of the study population and the associated lag time between the nutritional assessment and measurement of the REE by IC was as follows: 0 days (11%), 1 day (20%), 2 days (16%), 3 days (11%), 4 days (19%), 5 days (8%), 6 days (8%), and 7 days (7%). The subset population (N = 34, 31% of the study population) that we focused on to perform additional analysis was limited to a lag time of 1 calendar day or less to decrease patient condition variance.
Table 1 identifies the patient–specific characteristics for the study population based on the 2 study cohorts. A Pearson product–moment correlation showed a statistically significant correlation between the REE and EEE for our study population (N = 108, R = 0.802, P ≤ 0.001). The REE trended lower than the EEE values (Figure 2). The median difference between the REE and the EEE was 259 kcal/day with an IQR of –458 to –114 kcal/day.
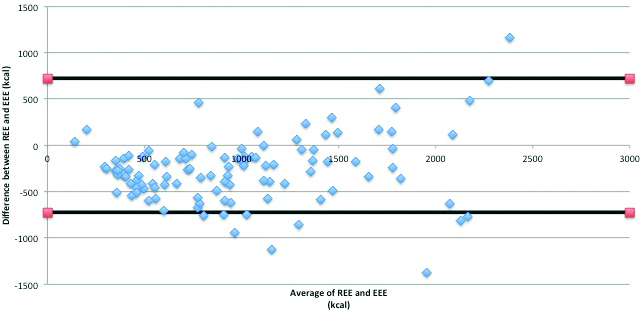
Total (n = 108) Median (IQR) | Subset (n = 34) Median (IQR) | |||
---|---|---|---|---|
Demographics | Age (years) | 6.0 (1.5-14.0) | 9.0 (2.8-15.3) | |
BMI | 17.6 (14.6-21.9) | 18.9 (15.7-24.3) | ||
Weight (kg) | 20.5 (9.4-46.0) | 37.5 (9.0-60.3) | ||
Energy estimates | REE | 684.5 (327.3-1219.0) | 875 (368.3-1456.8) | |
EEE | 1091.5 (711.8-1416.8) | 1109.0 (677.5-1400) | ||
Respiratory quotient | 0.85 (0.78-0.93) | 0.84 (0.75-0.92) | ||
Severity scores | PRISM 3 | 7.0 (2.0-12.0) | 7.0 (2.8-12.0) | |
PRISM 3 Probability death | 1.8 (0.5-5.5) | 2.1 (0.4-4.3) | ||
Vital signs | Systolic BP (mmHg) | 106.0 (90.5-124.0) | 115.0 (95.0-128.3) | |
Diastolic BP (mmHg) | 56 (48.0-67.0) | 60.0 (44.8-72.8) | ||
Heart rate (bpm) | 104.0 (93.0-126.0) | 98.0 (86.3-125.8) | ||
Temperature (°C) | 37.0 (36.6-37.6) | 37.0 (36.6-37.6) | ||
Respiratory support | FiO2% during IC | 35.0 (30.0-40.0) | 30.0 (30.0-40.0) | |
Tidal volume (mL/kg) | 106.0 (54.0-250.0) | 185.0 (59.3-387.5) | ||
Respiratory rate (breaths/min) | 20.5 (15.0-30.0) | 18.0 (12.0-30.5) | ||
PEEP (cmH2O) | 6.0 (5.0-8.0) | 5.0 (5.0-8.0) |
Table 2 displays factors that were associated with the observed difference between the REE and EEE. Of the 12 covariates tested in our linear regression analysis, we identified 3 that were independently associated with the observed difference. Those 3 covariates were age, heart rate, and tidal volume (p = 0.028, p = 0.000, and p = 0.044, respectively). Our analysis demonstrates that 57% of the observed variability is associated with these 3 covariates (P < 0.05; R2 = 0.565). Factors not associated included weight, BMI, severity scores (PRISM III), vital signs (temperature, systolic blood pressure, diastolic blood pressure), and mechanical ventilator settings (FiO2, frequency, PEEP).
Covariates | Unstandardized | Coefficients | Standardized Coefficients | t | Sig. |
---|---|---|---|---|---|
B | Std. Error | Beta | |||
Age | 18.474 | 7.880 | .469 | 2.344 | .028 |
Heart rate | 8.139 | 1.841 | .769 | 4.421 | < 0.001 |
Tidal volume | .704 | .331 | .482 | 2.123 | .044 |
Conclusion
Through the past several decades, the use of IC has consistently shown a differing estimation in energy expenditure from a variety of equations in both pediatric and adult populations.[11],[20],[27]–[29] These equations have been the focus of many validation studies, with no single equation showing complete universality; however, situation–specific equations have been shown to be reliable.[9],[11],[15],[17],[27]–[30] While IC is the preferred method of energy estimation for its higher degree of accuracy, it remains costly in terms of time, equipment, and the need for specially trained personnel.[27],[31]–[33] We believe that further testing with IC can be used to identify factors that contribute to the observed difference between current nutritional equations and IC. Identification of these factors may allow for the adaptation of more detailed and accurate nutritional assessment equations.
Unique to our study, one of our goals was to examine specific factors that may influence the observed difference between measured and predicted REE. To begin with, we found no previous literature that tested for specific factors to explain the discrepancy between estimated and measured energy expenditure in PICU populations; however, others have speculated on sources of discrepancies.[16],[20],[28],[34]
Martinez et al (2004) discusses numerous factors that contribute to the overall metabolic activity of an individual, especially one in the ICU, including factors at the time of admittance and conditions at the time of nutritionist estimation and IC completion. Those factors have been incorporated into nutritional equations under categories such as stress, growth, and repletion factors, yet these are still just estimations, which may not necessarily represent the true state of each unique individual. In our study, we attempted to take into account more specific metabolic factors during testing; we found 3 factors that contributed to 57% of the discrepancies between the equation estimate and IC. An array of other researchers also discuss the equations in question having been formed through validating one specific population, while the extrapolation to other populations is inaccurate.20,28,34 Currently, there are no equations that take race into account when estimating energy, which may be of consequence.[34]
In order to better adapt the current plethora of nutrition equations to a higher accuracy, a great amount of individual patient factors should be taken into account. We observed a number of factors, as seen in Table 2, looking for a significant variable that may lead to an effect on energy estimation. Our study suggests that clinical nutritionists should evaluate the vital signs, age, and tidal volume of the patient at the time the IC test is performed when comparing results of an energy estimate equation and IC test. Further research into the accuracy of modified estimations utilizing age, heart rate, tidal volume, and race is necessary to advance the treatment of critically ill populations.
Our current study was limited by several factors, however, primarily due to the study being conducted as a convenience study. Indirect calorimetry testing was completed with respect to convenience of staff and consenting patients and families; however, we still required a 24–hour limit on the time between IC completion after the clinical nutritionists estimations for our subset population. Over the 34–month data collection, 19 clinical nutritionists had performed patient assessments in our aggregate population of 108 patients, with the equation, formula, stress factors, and so on based on their best clinical judgment as nutritionists. There is variability in the assessments used because no single predictive formula is applied across the board; every nutritionist has a certain amount of formulas they can refer to. The formulas used are based on the clinical expertise and bias of the nutritionist. With multiple clinicians, over– or underestimation is likely to be more prevalent in one individual compared to another, leading to variation among EEE values. We made no effort to limit nutritional assessment to an individual nutritionists, as the 19 clinicians involved represent real–world conditions at our institution. We also acknowledge a final limitation of our study being that neither the individual’s race nor his or her sedation level was taken into account, affecting the resting energy state of our population.[34],[35]
Our study demonstrates that a statistically significant difference in energy expenditure as measured by IC (REE) compared to an estimate by a clinical nutritionist (EEE). In addition, we have demonstrated that if the lag between nutritionist assessment and IC performance is equal to 1 calendar day or fewer, 57% of the observed difference between the REE and EEE is attributable to the patients’ age and heart rate, as well as the tidal volume setting on the mechanical ventilator at the time the IC test is performed. Therefore, clinical focus on aspects of the patient’s condition, such as vital signs, ventilator settings, and age, are important when comparing IC and standardized equations for estimating energy expenditure. Further testing to identify additional covariates is suggested, as it may help elucidate the relationship between measured and estimated energy expenditure.
References
Pollack MM, Ruttimann UE, Wiley JS. Nutritional depletions in critically ill children: associations with physiologic instability and increased quantity of care. J Parenter Enteral Nutr. 1985;9(3):309–313.
Mehta NM, Duggan CP. Nutritional deficiencies during critical illness. Pediatr Clin N Am. 2009;56:1143–1160.
Mehta NM, Bechard LJ, Cahill N, et al. Nutritional practices and their relationship to clinical outcomes in critically ill children–an international multicenter cohort study. Crit Care Med. 2012;40:2204–2211.
Hulst JM, Joosten K, Tibboel D, et al. Causes and consequences of inadequate substrate supply to pediatric ICU patients. Curr Opin Clin Nutr Metab Care. 2006;9:297–303.
Briassoulis G, Zavras N, Hatzis T. Malnutrition, nutritional indices, and early enteral feeding in critically ill children. Nutrition. 2001;17(7/8):548–557.
Hulst JM, van Goudoever JB, Zimmerman LJI, et al. The effects of cumulative energy and protein deficiency on anthropometric parameters in a pediatric ICU population. Clin Nutr. 2004;23:1381–1389.
Coss–Bu JA, Klish WJ, Stein F, et al. Energy metabolism, nitrogen balance, and substrate utilization in critically ill children. Am J Clin Nutr. 2001;74:664–669.
Tappy, L. Metabolic consequences of overfeeding in humans. Curr Opin Clin Nutr Metab Care. 2004;7(6):623–628.
de Neef M, Geukers VGM, Dral A, et al. Nutritional goals, prescription and deliver in a pediatric intensive care unit. Clin Nutr. 2008;27:65–71.
Mehta NM, Bechard LJ, Leavitt K, et al. Cumulative energy imbalance in the pediatric intensive care unit: role of targeted indirect calorimetry. J Parenter Enteral Nutr. 2009;33(3):336–344.
Taylor RM, Cheeseman P, Preedy V, et al. Can energy expenditure be predicted in critically ill children? Pediatr Crit Care Med. 2003;4(2):176–180.
Rogers EJ, Gilbertson HR, Heine RG, et al. Barriers to adequate nutrition in critically ill children. Nutrition. 2003;19:865–868.
Kyle UG, Jaimon N, Coss–Bu JA. Nutrition support in critically ill children: underdelivery of energy and protein compared with current recommendations. J Acad Nutr Diet. 2012;112:1987–1992.
Mehta NM, Bechard LJ, Dolan M, et al. Energy imbalance and the risk of overfeeding in critically ill children. Pediatr Crit Care Med. 2011;12:398–405.
Coss–Bu JA, Jefferson LS, Walding D, et al. Resting energy expenditure in children in a pediatric intensive care unit: comparison of Harris–Benedict and Talbot Predictions with indirect calorimetry values. Am J Clin Nutr. 1998;67:74–80.
Martinez JLV, Martinez–Romilla PD, Sebastian JD, et al. Predicted versus measured energy expenditure by continuous, online indirect calorimetry in ventilated, critically ill children during the early post injury period. Pediatr Crit Care Med. 2004;5(1):19–27.
Cooney R, Frankenfield D. Determining energy needs in critically ill patients: equations or indirect calorimeters. Curr Opin Clin Care. 2012;18(2):174–177.
Meyer R, Kulinskaya E, Briassoulis G, et al. The challenge of developing a new predictive formula to estimate energy requirements in ventilated critically ill children. Nutr Clin Pract. 2012;27(5):669–676.
Wines K, Rzepecki A, Andrews A, et al. Validation of the V(max) metabolic cart in a simulated pediatric model. J Parenter Enteral Nutr. 2015;39(3):353–358.
Frankenfield D, Roth–Yousey L, Compher C. Comparison of predictive equations for resting metabolic rate in healthy nonobese and obese adults: a systematic review. J Am Diet Assoc. 2005;105(5):775–789.
Rao ZY, Wu XT, Liang BM, et al. Comparison of five equations for estimating resting energy expenditure in Chinese young, normal weight healthy adults. Eur J Med Resc. 2012;17:26.
Kim MH, Kim JH, Kim EK. Accuracy of predictive equations for resting energy expenditure (REE) in nonobese and obese Korean children and adolescents. Nutr Res Pract. 2012;6(1):51–60.
Weijs PJ, Vansant GA. Validity of predictive equations for resting energy expenditure in Belgian normal weight to morbid obese women. Clin Nutr. 2010;29(3):347–351.
SensorMedics. Vmax Operator’s Manual. Yorba Linda, CA: SensorMeds; 2002.
Smyrnios NA, Curley FJ, Shaker KG. Accuracy of 30–minute indirect calorimetry studies in predicting 24–hour energy expenditure in mechanically ventilated critically ill patients. J Parenter Enteral Nutr. 1997;21:168–174.
Smallwood CD, Mehta NM. Accuracy of abbreviated indirect calorimetry protocols for energy expenditure measurements in critically ill children. J Parenter Enteral Nutr. 2012;36:693–699.
White M, Shepherd RW, McEinery JA. Energy expenditure in 100 ventilated, critically ill children: improving the accuracy of predictive equations. Crit Care Med. 2000;28(7):2307–2312.
Flanchabum L, Choban PS, Sambucco S, et al. Comparison of indirect calorimetry, the Fick method and prediction equations in estimating the energy requirements of critically ill patients. Am J Clin Nutr. 1999;69:461–466.
Reid CL. Poor agreement between continuous measurements of energy expenditure and routinely used predictions equations in estimating the energy requirements of critically ill patients. Clin Nutr. 2007;26:649–657.
Sion–Sarid R, Cohen J, Houri Z, et al. Indirect calorimetry: a guide for optimizing nutritional support in the critically ill child. Nutrition. 2013;29:1094–1099.
Mehta NM, Compher C, A.S.P.E.N Board of Directors. A.S.P.E.N. clinical guidelines: nutritional support of the critically ill child. J Parenter Enteral Nutr. 2009;33(3):260–276.
American Society for Parenteral and Enteral Nutrition. The A.S.P.E.N. pediatric nutrition support core curriculum. 2010.
Kyle US, Arrisoa A, Esposity M, et al. Is indirect calorimetry a necessity or a luxury in the pediatric intensive care unit? J Parenter Enteral Nutr. 2012;36(2):177–182.
McDuffie JR, Adler–Wailes DC, Elberg J, et al. Prediction equations for resting energy expenditure in overweight and normal–weight black and white children. Am J Clin Nutr. 2004;80(2):365–373.
Vernon DD, Wittle MK. Effect of neuromuscular blockade on oxygen consumption and energy expenditure in sedated, mechanically ventilated children. Crit Care Med. 2000;28(5):1569–1571.