Understanding the Dynamics of Co-creation of Knowledge
This work is licensed under a Creative Commons Attribution-NonCommercial-NoDerivatives 4.0 International License. Please contact : [email protected] to use this work in a way not covered by the license.
For more information, read Michigan Publishing's access and usage policy.
Abstract
Community-campus engagement (CCE) offers transformative opportunities for collaborative knowledge creation. Over the last few decades, thoughtful energy has gone into identifying the parts of a CCE and then developing tools to study these parts, with discrete focus on community groups, students, and faculty. Bringing a complexity science approach to CCE evaluation can amplify our understanding by capturing the dynamic nature of collaborative interactions that occur between faculty and students and their relationship with community organizations and groups. Our article begins by introducing key features of a complexity science approach that are well suited to address the evaluation of CCE initiatives. We then position CCE within this approach and discuss how key features of complex systems can operate in the context of CCE. These features include a focus on context and initial conditions, dynamic interactions of adaptation and learning that are nested at different scales, and outcomes that emerge and self-organize in unexpected and nonlinear ways. We draw upon the contextual fluidity (CF) practice model that provides a bridge between abstract concepts of complexity science and the very practical world of community engagement. With awareness of context, dynamic interactions, and emergent outcomes, we propose questions that those evaluating CCE may want to consider.
Introduction
Community-campus engagement (CCE) creates a potentially transformative nexus for the exchange and diffusion of both academic knowledge and the place-based knowledge of community groups (Berinyuy et al., 2014; Bushouse, 2005; Enos & Morton, 2003). Higher education institutions have historically been the hubs for both knowledge creation and dissemination (Nelson & Stroink, 2012; Tremblay, C., Singh, W., & Lepore, W., 2017a; Tremblay, C., Spilker, R., Nagel, R., Robinson, J. C., & Brown, L. 2017b). CCE offers transformative opportunities for the co-creation and co-dissemination of knowledge through interactive collaborations between higher education institutions (faculty, students, staff, and administration) and community groups (Boyer, 1996). Local, place-based knowledge of community groups can enhance the insights and potentially the applicability of knowledge within context (Siemers, Harrison, Clayton, & Stanley, 2015). CCE promotes the democratization of knowledge sources and offers an accessible and multidisciplinary perspective (Tandon, Singh, Clover, & Hall, 2016; Brown et al., 2003). CCE can also assist in capacity building of community groups (Andrée et al., 2014). Evaluating CCE initiatives in a way that can capture the fullness of such potential impacts presents unique challenges (Reeb, et al., 2017; Stoecker, Beckman, & Min, 2010). We suggest that understanding CCE through the perspective of complexity science offers insight into its dynamics and raises questions that can guide approaches to its evaluation.
Although CCE may take different forms, including community service learning (CSL), community-based research (CBR), and participatory action research (PAR), we focus in this article on evaluation with CCE as a general term capturing the dynamic nature of the collaborative interactions that occur between faculty and students and their relationship with community organizations and groups. In our experience, many CCEs combine different forms such as CSL with CBR or PAR with CBR (Kahlke & Taylor, 2018). Both authors engaged in a university-wide community service learning initiative that involved 28 faculty members from seven academic faculties with participation of students from 16 diverse disciplines organized around the theme of food security or sustaining access to food. This themed approach to CCE encouraged faculty, staff, and student participation with diverse community groups and organizations ranging from government to food banks to First Nation communities and resulted in the emergence of diverse new courses such as Food Issues in Northern First Nations. This CCE nexus resulted in a variety of research publications (e.g., Nelson & Stroink, 2010, 2012; Stroink & Nelson, 2009, 2013). Our article builds on decades of research on CCE (e.g., Munz, Gatchet, & Meier, 2018) as well as on a growing body of critical approaches to evaluation (e.g., Patton, 2011). To this work we introduce and weave in our analysis of complexity science as a framework for conceptualizing community-campus engagement.
There have been a few evaluation models specifically developed that document the impacts of community-engaged scholarship (Reeb et al., 2017; Stoecker et al., 2010). Models such as cause and effect and logic models (Kania & Kramer, 2013) have been constructed on a positivistic lens for evaluation and are based on assumptions that may not hold in the dynamic context of CCE. These assumptions include (a) that by digging into the parts of a collaboration, we can explain and assess the whole; (b) that relationships are simple cause and effect; (c) that the future is knowable if there is enough data; and (d) that prediction and control of the future are possible (Malinsky & Lubelsky, 2014; Preskill, Gopal, Mack, & Cook, 2014). We build on other critical approaches to program evaluation to support the need for a different approach for evaluating CCE initiatives that challenges such “Newtonian” machine-age thinking (e.g., Patton, 2011; Pawson & Tilley, 2004; Smits & Champagne, 2008). We argue that an approach rooted in complexity science accounts for the unique characteristics of CCE where the relationships are dynamic, multi-directional, and nonlinear and where sensitivity to initial conditions can bring forth unanticipated outcomes.
There is a long history of focus on caring about and sensitivity to the impact of context on program evaluation (Denizen, 2009; Greene, 1994; Lincoln & Guba, 1985; Patton, 2015, 2008). Multiple stakeholders, the recognition that all evaluation occurs within a political context, and the impact of the evaluator on the evaluation have all been valuable contributions to understanding the key role of context and emergent outcomes. Likewise, the use of positivistic approaches has given us great insights into the functioning of a CCE. In our own research with CCE and food security and food systems issues, we have benefited from these contributions e.g. (Nelson & Stroink, 2010). Our journey with CCE and complexity science has made us aware of how a complexity approach illuminates the interacting elements that make a CCE a complex system with unique and emerging outcomes. As we explain in more detail below, when envisioning a CCE as a complex system, we can build rich insights as to how the independent and unpredictable actions of each component of a complex system such as the faculty, students, staff and organizations or groups changes the context of each component. Thus, a complexity approach adds to a CCE evaluation through consideration of the pattern dynamic and ever adapting nature of a CCE. Through our hands-on years of engaging a higher education institution in multiple CCEs we have come to appreciate how a complexity approach enhances our understanding of “what is going on” in a CCE.
Our article begins by introducing key features of a complexity science approach that are well suited to address the evaluation of CCE initiatives. We then position CCE within this approach and discuss how key features of complex systems can operate in the context of community-campus engagements. These features include a focus on context and initial conditions, dynamic interactions of adaptation and learning that are nested at different scales, and outcomes that emerge and self-organize in unexpected and nonlinear ways. To bridge the theoretical space of complexity science with practical questions of CCE evaluation, we then summarize the contextual fluidity (CF) practice model (Andrée et al., 2014; Nelson & McPherson, 2004). Drawing on this model, we then propose a set of guiding questions that enable a more complete view of CCE by exploring their features as complex systems.
Complexity Science Approach
This article approaches understanding of campus-community engagement with a complexity science perspective. Complexity science is a multidisciplinary meta-theory and arguably an alternative epistemological paradigm of science itself (Guastello & Liebovich, 2009). Complexity science offers conceptual and methodological tools for understanding phenomena that emerge from the interactions of many interdependent agents in ways that are not always predictable or reducible to linear causal sequences. This approach and complex adaptive systems theory specifically are useful in understanding phenomena that change over time, adapting continuously to a dynamic environment or context (Nicolis & Prigogine, 1989; Waldrop, 1992; Walker & Salt, 2006). Social groups and communities have been described and studied as complex adaptive systems (Masterson et al., 2017; Plexus Institute, n.d.; Westley, Zimmerman, & Patton, 2006). A complex system occurs where a collection of independent, yet interdependent agents or parts interact with one another and with their context and through these interactions produce outcomes that are not predictable from or reducible to the behavior of the parts (Holling, 2001; Page, 2015). In complex adaptive systems, feedback loops further allow the system as a whole to adapt and learn (Holland, 1992, 2006). A defining quality of these systems is emergence, which describes the formation of complex, organized, system-wide patterns of behavior through local interactions among agents guided only by a set of simple rules. The emergent behavior self-organizes in that there is no centralized planning or control producing the pattern (Kauffman, 1995; Moore, Olsson, Nilsson, Rose, & Westley, 2018; Piers, 2007). In a community, the individual members are the agents, each of whom is free to act in unpredictable ways. However, they are interdependent through their connections with one another. As each one acts, they affect the behavior of the others and thus also affect their own context, to which they must again adapt. In this way, and by following simple rules such as reciprocity and other social norms, patterns of community-level outcomes emerge (Manson, 2001; Mitchell, 2009). Higher education institutions may also be viewed as complex adaptive systems, akin to businesses and other organizations that have been extensively studied through this lens (Burnes, 2005; Burnes, Hughes, & By, 2018; Dooley, 1997).
In a higher education institution, students, faculty, and staff interact with one another following simple rules that include their various roles and norms. These simple rules provide continuity and can be changed with varying amounts of ease as the agents adapt dynamically to one another and to the particular space and time. In this way, the relationships among the agents within the system become self-organized in an emergent pattern. These patterned relationships serve to coordinate the system’s functions and the organization and re-organization of the university over time is thus an example of emergence (Goldstein, 2008).
At this point, we need to introduce two additional concepts from complexity science that will aid in explaining how higher education institutions and communities, both as complex systems, interact with each other. Complex systems change over time in an adaptive cycle (Figure 1). In the first phase of this cycle, termed growth, initially chaotic conditions give way to increasing structure and organization that emerge in the second phase, termed conservation. This structure and organization facilitate the adaptation of the system and enhance its efficiency and stability. Over time, the maintenance of that structure consumes the majority of the resources (e.g., time, energy) available to the system. The system is then rigid and unable to respond quickly to changes in its context. In the third phase, termed release, a triggering event can cause some or all of the system’s organization to be released in a collapse, and its behavior returns to a more chaotic state. From here, the resources and materials of the system in their context are reorganized in a fourth phase called reorganization, which is characterized by experimentation. When there are sufficient resources present to support the self-organization of an emerging adaptive system formation, a new phase of growth occurs (Kauffman, 1993). This cycling from organization through release allows the system to remain adaptive to its environment while introducing novelty. Research in forest sciences (Gunderson & Holling, 2002; Holling, 2001) and business and organizational studies supports this proposition (Mitleton-Kelly, 2003; Stacey, 1996; Uhl-Bien, Marion, & McKelvey, 2007). More recently, there is interest in how the adaptive cycle can enhance an understanding of dynamic nonlinear relationships in international community engagement service learning (Berinyuy et al., 2014).
Complex systems are also arrayed on multiple scales, and the adaptive cycles of systems are interconnected across different scales in ways described by Gunderson and Holling (2002) and termed panarchy (Figure 1). In a panarchy, larger and slower cycling systems (e.g., provincial regulations governing higher education institutions) contain smaller and faster cycling systems (e.g., higher education institutions) that in turn contain still smaller and faster cycling systems (e.g., academic departments). As a system self-organizes, its processes are influenced by the structures of the next higher scale, a phenomenon called remembering. Remembering processes can act to stabilize the overall system as larger, slower cycles maintain and perpetuate system patterns in the smaller, faster cycles nested below. Likewise, a system undergoing release or collapse can at times trigger a cascading release in a linked system at the next higher scale in a process called revolt. The remembering process can act like a brake, dampening down and containing the effects of revolts. In the concepts of the adaptive cycle and panarchy we can see that systems are dynamic and interconnected in nested scales.
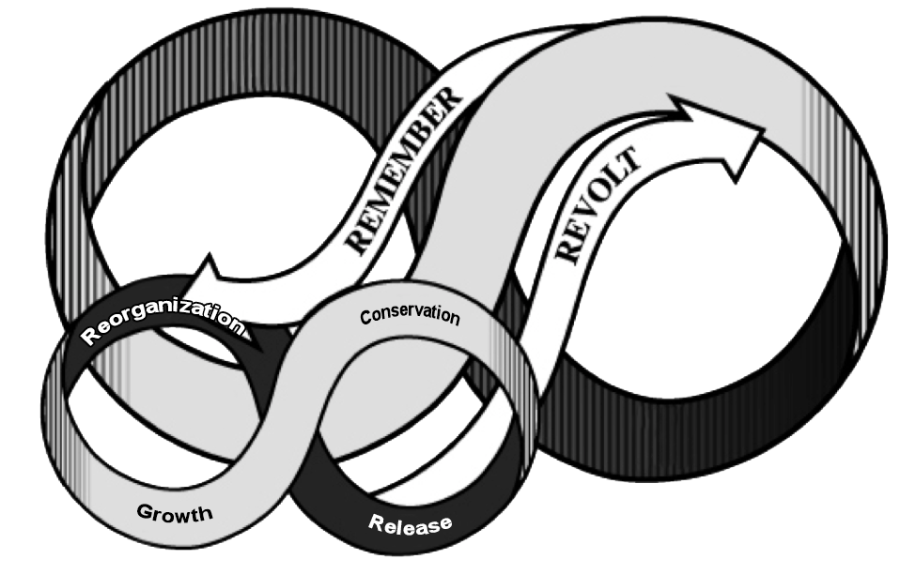
Adaptive cycles and panarchy scales can be found in both higher education institutions (e.g., Aldrich, 2015; Forsman, Linder, Moll, Fraser, & Andersson, 2014) and communities (e.g., Westley et al., 2006). Furthermore, the university, as a local employer and service provider, is in some ways nested within its geographic community, and both can be influenced by each other’s adaptive cycles. Also, when examined closely, the boundaries differentiating university and community are fuzzy and contextual as opposed to fixed and permanent. For example, the agents comprising the university system are also agents within the community system; where the boundary lies may sometimes depend on the thought processes of individuals. This latter insight supports the notion of conducting CCE from the perspective of “in community,” a concept to which we will return. We next explore how key features of a complexity science approach can inform our understanding of CCE as the co-creation of knowledge occurs. From there we draw on the CF framework to propose an approach to evaluation of CCE that can address the dynamics of the interactions at the center of the CCE as well as the unpredictable events and knowledge that can emerge from the whole.
CCE in a Complexity Science Approach
Over the last few decades a lot of thoughtful energy has gone into identifying the parts of a CCE and then developing tools to study these parts, with particular focus on community groups, students, and faculty as discrete groups (Janke, E.M., 2009; Munz et al., 2018; Olney & Grande, 1995; O’Meara & Niehaus, 2009; Worrall, 2007). Much has been learned about CCE and the co-creation of knowledge from this approach. However, an underlying assumption has been that a CCE as a whole is understandable through analysis of its parts. Less focus has been given to the dynamics that operate in between these parts and the outcomes that emerge from these interactions that are greater than the sum of the parts. Bringing a complexity science approach to CCE evaluation can amplify our understanding by orienting attention to the interactions among the parts, by providing ways to describe the effects of the CCE on a holistic level, and by shifting how we think of these parts in context. For the purposes of this article, we will focus on context and initial conditions; dynamic interactions of adaptation and learning that are nested at different scales; and outcomes that emerge and self-organize in unexpected and nonlinear ways.
In CCE from a complexity approach, the people who are the agents within the community entity and the university respectively are forging connections with each other that reach across the fuzzy boundaries of the community and university silos. The context and state of the first interactants set the initial conditions in which the collaboration begins. As the agents become interdependent, each adapts to their new context by changing their own behavior, that in turn changes the context and results in further adaptation by the other people involved. Through continued collaboration, a network of people in the CCE takes shape, and it becomes its own complex adaptive system, nested within both the community and university and interacting with its context. Community and academic partners immersed in the same environment and engaged together with the same issues in this way have been described as working “in-community” (Harrison, Nelson, & Stroink, 2013). Thus, the initial conditions in the people and their context, the nature of the interactions among the various people in a CCE, and the simple rules that shape those interactions are important in evaluating the dynamics at the heart of the CCE.
Through these dynamics, the CCE as a complex adaptive system generates emergent outcomes, patterns, and characteristic qualities that are greater than the experiences of the individual people or organizations within the system. These emergent outcomes may be nonlinear and unpredictable from the behavior of the individuals or parts of the whole, forming as they do as a result of the initial conditions and subsequent iterative effects of countless interactions. Furthermore, the nature of these emergent outcomes is fed back to the individuals or organizations through positive or negative feedback loops, thus enhancing or diminishing certain behaviors within the network. In this way, the system as a whole learns, and the CCE can be responsive to its environment. Emergent outcomes in a complex system are self-organizing, meaning that the dynamics of the system itself produces the outcomes, with no requirement for centralized planning and control. In CCE, the different skills and resources of the community participants interact with academic skills of analysis, reflection, and documentation to result in emergent outcomes that need not be controlled by anyone, either internal or external to the work, and are a dynamic product of continuous adaptation in place. Thus, in evaluating a CCE, in addition to examining the experiences of individuals, groups, or organizations within the CCE, it is important to also examine emergent outcomes on the level of the whole system, including unexpected events, interaction patterns, and any emergent system structures and learning.
One of the major emergent outcomes of CCE is knowledge. Nested within each of the individual agents in a CCE is a cognitive meaning and information system in which order or meaning continuously self-organizes based on embodied interactions among sensory information and cognitive processes occurring in a symbolic and generally social context (Hollis, Kloos, & Van Orden, 2009). In this way new knowledge can be said to emerge at the individual, group, organization, and social levels simultaneously, in self-similar patterns reminiscent of fractal patterning (Stacey, 2001). There is an extensive literature on learning in organizations (e.g., Lipshitz, Popper, & Friedman, 2002; Mishra & Bhaskar, 2011; Popper & Lipshitz, 2000; Stacey, 2001). One observation is that when an organization has networks of information exchange that include more diverse perspectives, the emergent knowledge tends to be more innovative and adaptive to context (Guillaume et al., 2014; Moore et al., 2018; Yang & Konrad, 2011). In CCE, knowledge is generated through interactions among more diverse agents and in more immediate adaptation with the community context than in typical academic scholarship.
As a complex adaptive system, a CCE initiative may also demonstrate some or all of the phases of the adaptive cycle and may be influenced by processes of revolt and remembering in the panarchy of systems in which it is embedded. As the initiative takes shape and enters the growth phase of the adaptive cycle, connections expand, and there is a diversity of opportunities and ideas that blossom. As the relational and intellectual capital of the initiative grows, there is an increasing need to organize that growth, which sees the formation or formalization of system structure into the conservation phase of the cycle. That structure, initially formed in adaptation with the context, may become less adaptive as the context changes, and a release of structure may occur. This may be experienced as disorienting and unpleasant as the initiative reorganizes. This cycle of growth, release, and reorganization has been studied extensively in the literature on organizational management (Mitleton-Kelly, 2003; Stacey, 1996; Uhl-Bien et al., 2007). One insight from this literature is that undergoing periodic releases before the system gets too deep into the conservation phase can increase innovation and resilience in the system (Biggs, Westley, & Carpenter, 2010).
The same adaptive cycle observed on the level of the initiative as a whole may also be seen in the thought and meaning systems of individuals within the initiative. As engagement with community issues and partners opens new areas of thought and awareness in the students, these new thoughts are given meaning through the formation of mental structures that are in part influenced by larger levels of meaning in the students’ panarchy of prior thoughts and beliefs. Over time, some of these mental structures are released and reorganized to make way for new thoughts that are better adapted to a changing learning environment (Gunderson & Holling, 2002). These thought system adaptive cycles are nested within the adaptive cycle of the initiative itself, which is in turn nested within the adaptive cycles of the higher education institution and community contexts. Processes of remembering and revolt can lead to cascading changes across levels between individuals or organizations and initiatives and beyond to the community and higher education institution levels.
Evaluation of CCE
We have learned much about CCE through studying the parts—community, faculty, and students (Astin, Vogelgesang, Ikeda, & Yee, 2000; Sandy & Holland, 2006; Seifer & Connors, 2007). For example, research using both quantitative and qualitative methods has identified (1) benefits for community groups including access to human resources and new ideas (e.g., Blouin & Perry, 2009), (2) benefits to students including increased learning of course material (e.g., Wickersham, Westenberg, Jones, & Cress, 2016) and civic participation (e.g., Nelson & Stroink, 2010), (3) faculty experiences with service learning (Clayton & O’Steen, 2010; Harrison et al., 2013; Miller-Young et al., 2015), and (4) typologies of faculty engagement (Morrison & Wagner, 2016). However, from a complexity science perspective in which a CCE is understood to be a complex system, it is clear that a comprehensive approach to evaluation requires analysis of context, dynamics, and emergent outcomes of the initiative. In addition to the independent parts (deductive positivist approach), we need to look at the dynamic interdependencies holding the parts together as well as at the resulting context-interdependent emergent outcomes. As previously discussed, a complexity science approach allows us to explain how the interactions of the independent agents can change the context.
In addition to an analysis of the parts, we suggest that a complexity science perspective evaluation should also (a) consider the context of the CCE, (b) examine the interactions themselves (Bandy et al., 2016), and (c) observe emergent outcomes (Osberg, Biesta, & Cilliers, 2008). Consideration of the context includes analysis of the initial conditions, the state of the first agents as they came together to interact, and the nature of and features surrounding those first interactions. The evaluation should also consider the state of the systems in which the CCE is nested and the diversity inherent in the context and agents in the initiative. Examining the interactions within a CCE includes exploring the simple rules or norms that guide the interactions, the diversity and scope of the network connections that form, the level of interdependence and distributed control that takes shape, and the dynamics of adaptation that occur over time. Observing emergent outcomes allows evaluation to reveal ways that the CCE is greater than the sum of its parts. This includes identifying patterns or emergent structures within the initiative, outcomes that did not exist before the initiative within any of its parts, recognizing that some of these outcomes may be unexpected and may have emerged in a nonlinear manner that is not predictable from or reducible to the behavior of the parts.
This complexity approach to CCE evaluation thus allows a switch in focus from questions about representation and presentation of various components of a CCE to questions of the engagement itself and responses to the engagement (Osberg et al., 2008). Viewing a CCE as a complex system adds immensely to the understanding of campus and community collaborations by finding the pattern dynamics in the whole system rather than zeroing in on distinct decoupled parts. The evaluation process from this perspective observes how through interdependence more can be achieved than ever could be done by a single entity. In the sections that follow, we explore how consideration of the context, dynamic interactions, and emergent outcomes can provide a more complete evaluation of CCE initiatives. To do so, we draw upon the CF practice model (Andrée et al., 2014; Nelson & McPherson, 2003, 2004; Nelson, Stadey, & Lyons, 2005). This model informs approaches to partnership and engagement and was the foundation for the food security–themed CSL initiative we launched with support from the J. W. McConnell Family Foundation. This model also draws on a complexity science perspective and provides a bridge between the abstract concepts of complexity science and the very practical world of community engagement.
Contextual Fluidity Practice Model
The CF practice model recommends approaches to partnership initiatives including community-university partnerships and engagement practices. The model includes five dimensions that act in concert. When each dimension is present and operating simultaneously in any order, the engagement’s capacity to realize adaptive and innovative outcomes is enhanced (Nelson & McPherson, 2004). These dimensions are vision, context, fluidity, webs of networks, and strange attractors. We will now provide a brief review of these five dimensions to inform our discussion of how CCE evaluation can incorporate consideration of the context, dynamic interactions, and emergent outcomes in the initiative. For more information on the CF practice model, see Nelson et al. (2005).
Vision. The CF approach to partnerships encourages the use of vision to guide the emergence of an initiative. A vision that is compelling, enlivens people’s sense of the possible, and is rooted in a set of shared values sets the simple rules for the engagement. Thus, the values and vision influence the very interactions out of which the initiative forms. A vision-driven initiative allows participants to be open and primed to jump into opportunities that emerge. A vision can guide the interactions in the CCE throughout the varying stages of the adaptive cycle while allowing the interactions themselves to be fluid and dynamic. This vision-driven approach is in contrast to approaches that emphasize a priori planning with fixed outcomes (Mintzberg, 1994). In these approaches, the outcomes may be as planned, but they are less likely to result in innovation (Westley & Antadze, 2010).
Context. The CF approach recognizes that interactions occur within both space and time as a dimension of place (Nelson & Stroink, 2014; Stroink & Nelson, 2013). Understanding the initiative in its full context ensures a detailed understanding of the initial conditions and avoids the assumption of one best approach. Recommendations include listening carefully, including for what is not said; minimizing us/them attitudes; engaging critics, as dissent often is a valuable measure of commitment (Block, 2009); and humor to facilitate trust and openness in relationships.
Fluidity. The CF approach embraces being fully present within the current context of an initiative. This fluidity encourages robustness, diversity, and flexibility in interactions, accepting incompleteness and contradictions in human behavior. The inevitability of ups and downs in interactions is embraced. Control is distributed, and leadership is facilitative, bringing forth the latent talents throughout the network of people comprising the initiative (Moore et al., 2018; Westley et al., 2013).
Web of Networks. The CF approach seeks opportunities for the ongoing, fluid expansion of formal and informal networks within and around the initiative. Such network interactions receive, relay, and interpret information. The various participants in the initiative may be members of more than one network but need not be members of all the networks. Vision is communicated and interpreted throughout the network enhancing diversity. Recommendations include the endorsement of multiple relationships, encouraging broad participation in the process, getting out of one’s comfort zone, and being careful that no one member shoulders too many responsibilities that might stifle others in participation and commitment.
Strange Attractors. The relationships within partnership initiatives conducted through the CF approach remain adaptable and open to novel and informal opportunities. These strange attractor sources of influence are not planned or anticipated and introduce diversity that increases adaptability within a complex system (Holland, 1998; Moore et al., 2018). This approach involves identifying and working with the sometimes-hidden forces or attractors that affect a system’s dynamics. Recommendations include being opportunistic in pulling in a diversity of resources.
The five dimensions of the CF practice model provide one image of what partnership initiatives look like when they are conducted with an awareness of the complex systems dynamics they contain. This bridges our efforts to explore the evaluation of CCE initiatives from a complex systems approach. In the next section we further explore this complex systems approach to evaluation of CCE initiatives by adding a focus on the context, dynamic interactions, and emergent outcomes.
Complexity Evaluation
Given the nature of CCE as a complex system, it is important to conduct evaluations with an approach that is able to capture these systems’ dynamics and outcomes. In classic approaches to evaluation, the goals and objectives for an initiative are articulated at the outset, and a plan is formed that is expected, based on prior research, to meet these objectives. This plan is then followed, without deviation, so that the behavior, attitudes, or knowledge of those involved in the initiative beforehand can be compared with their behavior, attitudes, or knowledge afterward, often also against a control group. Any observed changes are then taken as support for the principles by which the plan was formed, and the initiative is considered successful to the degree that it met the objectives articulated at the outset (Royse, Thyer, & Padgett, 2016).
Classic approaches to evaluation have their advantages (Bringle & Hatcher, 1996; Royse, et al., 2016; Smith, 2010). However, we build on the work of other critical approaches (Patton, 2011; Pawson & Tilley, 2004; Smits & Champagne, 2008) to argue that complex community initiatives such as CCE may not be fully or appropriately described by these classic approaches to evaluation. For example, Smits and Champagne (2008) argued for a participatory evaluation that goes beyond assessing the outcomes and worth of an activity. In the community context, participatory evaluation can be part of the social change agenda itself, as knowledge is co-constructed and translated into actionable decisions relevant in the local context. Pawson and Tilley (2004) and Patton (2011) describe approaches to evaluation that are suited to complex contexts. Pawson and Tilley (2004) argue that the outcomes of an initiative should be understood as patterns. These patterns form as the activities undertaken interact with a context that includes combinations of specific relationships, technology, economy, and places. These contextual factors interact with and determine the effectiveness of the activities in the program. Likewise, Patton (2011) argues for a developmental approach in which evaluation is ongoing and programs are allowed to adapt to their contexts as they run.
In each of these participatory and critical approaches to evaluation, learning is understood to be engaging, participatory, and ongoing. Initiatives or programs are viewed as contextual, with outcomes inseparable from the relationships and places in which they form. These approaches are in contrast to classic evaluation in which planning and learning are frontloaded and initiatives must run as planned, with the effectiveness of the plan assessed by the presence of the expected outcomes at the end.
Expanding on these approaches, drawing on the CF model of practice and community engagement, and using complexity science as the theoretical approach, we argue that evaluation should consider the context, the dynamics of interaction, and the emergent outcomes to fully evaluate CCE initiatives. In each of the following sections, we describe areas within an initiative that are not visible in classic approaches to evaluation and suggest some guiding questions that can help evaluators gain valuable information. Based on the authors’ CCE experiences, we briefly illustrate that evaluating these areas of context, dynamic interactions, and emergent outcomes can add fullness and depth.
Context
We suggest that evaluating the interactions that occur in a CCE can begin with examining the context with the agents of community, students, staff, and faculty. Both a CF model of practice and its theoretical basis in complexity science demonstrate that small differences and diversity can have a huge impact on emergent outcomes, adaptability, and innovation. We suggest that understanding the initial conditions of the various agents in a CCE can be helpful. Key questions to gauge initial conditions can include the following: What brought the various agents together in this CCE initiative? What were the first contacts like? What range of perspectives were brought in? And what was the state of the surrounding systems (what issues and conditions brought the community into the CCE, what were the higher education institution’s policies toward student enrollment practices and engaging with community)? Then as the CCE interactions continue, each of these independent agents become interdependent in their mutual and reciprocal learning. These ongoing interactions occur in a context that is also dynamic as articulated in the CF practice model (Nelson & McPherson, 2004). From the initial conditions, did this CCE initiative adapt to any changes in context? How did the higher education institution’s policies toward community engagement impact the CCE? How did the history of the community impact the initiative? How did the learning that occurred in the CCE absorb the elements of place?
Dynamic Interactions
In a CCE, both community partners and the higher education institutions are immersed in the same environment and together are engaged with the same issues. What are the simple rules and norms guiding the interactions? Were these rules shaped by vision and values as recommended by CF? Were us/them distinctions minimized as recommended by CF? As the CCE continues, what patterns of interdependence can be recognized?
A casual scan of CCE may give the appearance of many simultaneous and nonlinear events happening in a chaotic fashion. A complexity science approach brings an understanding to dynamic multi-directional tasking among community and campus groups by conceptualizing adaptive cycles as occurring across scales, whereby feedback loops encourage adaptive learning. When interacting “in community,” can the feedback loops be identified that demonstrate adaptation? Are there patterns of release and innovation as per adaptive cycle? We further suggest that these dynamic interactions of adaptive cycles nested one within the other across space and time can be understood by an evaluation focus on certain questions. For example, is there evidence of large, slow moving scales at the policy levels in both the higher education institution system and the community systems? What smaller, faster moving levels of CSL or CBR provide evidence of adaptive learning? What interactions moving across scales in adaptive cycles of learning can be identified? Are there interactions across scales that demonstrate revolt or remembering?
In a CCE, there is an opportunity for many formal and informal networks to emerge. Do the networks embrace a shared vision and values that provide connectivity among a variety of networks or community groups engaging in a CCE? What mechanisms does the CCE use to endorse multiple relationships across community groups that can encourage innovation? Does the CCE encourage the various agents to push out of their familiar context in order to expand networks in a CCE?
Control is distributed in a complex system. Is there evidence of leadership that facilitates a discovery approach to a CCE rather than selecting all the resources that will be used in the CCE? Is there evidence of encouraging students and community networks and participants to contribute resources, participate in setting agendas for the interactions, and encourage finding novel questions and solutions for the CCE experience? Is there evidence of facilitative leadership that encourages interactions among the participants that brings forth latent talents that spur the CCE into activities that could not have been brought forth through a prior planned process? Is there evidence that through distributed control unique and diverse voices are heard?
Is there evidence that the dynamic interactions encourage fluidity that offers opportunities to learn from mistakes and then move on, engages the unique gifts of all participants, and accepts as part of complex interactions incompleteness and contradictions in human behaviors?
Emergent Outcomes
When the independent agents interact and become interdependent in a CCE, some outcomes are unexpected, emerging in a nonlinear manner that is not predictable. Emergent outcomes in CCE go beyond assessments of the parts, such as whether the community met its goals through the project or whether the students learned their course material more effectively. Emergent outcomes could include new ideas, new projects, new knowledge, and new relationships. Some of these could not have been anticipated at the start of the initiative, as they emerged through interactions that depended heavily on the initial conditions of the project. These emergent outcomes further influence the way the parts interact through feedback loops, resulting in further unanticipated outcomes. Typically, evaluation focuses on whether the CCE met its initial goals. We suggest that it also look for unexpected emergent outcomes, including new knowledge.
For example, over several projects, community, faculty, and students engaged together around local, land-based production of food. Through these interactions, new knowledge emerged regarding approaches to food acquisition that blended cultivated and forest food practices in novel ways. This emergent knowledge was not gained by any one of the three groups but rather emerged through their interactions over time (Stroink & Nelson, 2009).
In seeking information on emergent outcomes, guiding questions for an evaluation can include the following: What unexpected outcomes emerged that were greater than the preplanned CCE objectives? What was the pattern of interaction that formed emergent outcomes? What evidence is there in the CCE that knowledge is viewed as more than acquired knowledge that adds to what is already known? One of the emergent outcomes in a CCE may be knowledge itself. What evidence is there that knowledge may evolve as a response to the interactions themselves and thus emerge as greater than the sum of the parts? What unexpected relationships formed? Are there examples of emerging structures that provide continuity past the time frame of the CCE?
Discussion
In this article, we reviewed complexity science as a meta-theory, along with concepts from complex adaptive systems theory, and then positioned community and higher education institutions as well as CCE in this complexity science approach. Through a brief review of the CF practice model, that is itself based in complexity science, we were able to present an image of CCE as a complex system. Through this review, we identified the need for CCE evaluation to consider the roles of context, dynamic interactions, and emergent outcomes. Consideration of context includes being aware of how small differences in the initial conditions of an initiative as well as the diversity of perspectives and features of the higher education institution and community contexts can influence its outcomes. Being aware of the dynamics of interaction at play in an initiative orients us to the simple rules or norms shaping interactions, the adaptive cycles of change that occur within and around the initiative, and approaches to control and leadership that may occur. Understanding how outcomes emerge from complex systems reminds those evaluating CCE to look for unexpected outcomes, including new knowledge, new ideas, and new initiatives that may not have been planned at the outset and may not be reducible to the perspectives of the individual separate parts of the initiative. With awareness of context, dynamic interactions, and emergent outcomes, we suggested questions that those evaluating CCE may want to consider as they conduct their evaluation.
Although we suggested questions that may serve as a guide to future evaluations of CCE from a complexity science perspective, we did not review specific methods or tools to use. Some promising methods can include observations, interviews, focus groups, Appreciative Inquiry, media tracking, and mapping exercises to collect evaluation data (Preskill et al., 2014, p. 25). Some recent tools that seem suitable when carrying out a CCE evaluation from a complexity science approach are noteworthy. For example, Outcome Harvesting has proven useful as an evaluation tool when outcomes occur in a dynamic and uncertain context in which the relation between cause and effect is complex (Wilson-Grau, 2015). The “most significant change” (MSC) technique encourages those involved in a project to relate stories of significant changes. These stories are collected and reviewed by staff or other stakeholders for stories that capture impact. Once a group of stories has been identified, the stories are read aloud, and discussions ensue about the value of impact changes that have occurred (Davies & Dart, 2005). In a CCE, the MSC technique may facilitate the identification of unplanned interactive outcomes. Likewise, ripple effect mapping (REM) is a useful tool for capturing impacts of unplanned outcomes (Chazdon, Emery, Hansen, Higgins, & Sero, 2017; Earl, Carden, & Smutylo, 2001; Hardy, Hulen, Shaw, Mundell, & Evans, 2018; Zimmerman et al., 2019). As explained previously, the participants in a CCE are free to act in unpredictable ways. Simultaneously, they are interdependent through their interactions with one another. As each one acts, they affect the behavior of others, thus affecting their own context to which they must again adapt. This cyclical adaptation process creates patterns of unplanned outcomes that may be captured through these tools. Future work can further develop these tools for the CCE context.
These promising new tools notwithstanding, one limitation in applying the complexity science perspective is the difficulty involved in gathering some of the required information. For example, the initial conditions of a CCE may have occurred years prior and would be subject to reinterpretation by any of the individual agents who are still involved. In addition, one potential risk in adopting a complexity science perspective is that researchers trade an exclusive focus on discrete and decontextualized parts for an analysis of contextualized dynamics that obfuscates what could be a simple linear causal relationship. As indicated throughout our analysis, we recommend balancing the strengths of a reductionistic approach with those of a complexity science approach.
Conclusion
A complexity science approach provides a unifying framework for evaluating CCE that emphasizes context dependency. Much of our evaluations on the parts of a CCE is based on traditional methodologies that spend enormous amount of effort zeroing in on understanding a part based on the belief that when we understand well one example in a specific context, we will understand how other CCEs operate in relation to the part studied. As previously stated in the introduction, studying the parts can provide valuable insight. However, CCEs are context dependent, and the initial conditions that surround a particular part are not easily transferred to that same part in another CCE.
Therefore, a complexity science approach to CCE evaluation provides a deeper and more comprehensive understanding because CCE processes are complex systems that can be shown to follow an adaptive cycle, display emergence, and operate at different panarchical scales within a nested system of adaptive cycles. We invite further discussion of what it means to consciously introduce a complexity science approach into evaluations of CCE. How can we deepen our learning about the dynamics of CCE by a holistic approach to evaluation of CSL? How can we sharpen our comprehension of the evaluation process when we observe the interactive patterns in CSL?
Acknowledgments
Our journey began with receiving one of the 10 J. W. McConnell Family Foundation Grants to educational institutions to study the research question of how a university can enhance their engagement with community through community service learning and community-based research (2006–2012). Through this experience, we became more intrigued with a complexity science approach and its potential benefits to understanding the dynamics in engagement among universities and community.
Our community service learning experiences have been bolstered by our relationship with Community First: Impacts of Community Engagement (CFICE).
A special thank you to all the community groups, students, and faculty with whom we have collaborated in delivering community service learning courses. We also wish to thank all of our students who have taken our complexity science seminar and utilized these concepts in carrying out community-based research.
References
- Aldrich, P. R. (2015). The curriculum prerequisite network: Modeling the curriculum as a complex system. Biochemistry and Molecular Biology Education, 43(3), 168–180.
- Andrée, P., Chapman, D., Hawkins, L., Kneen, C., Martin, W., Muehlberger, C., … & Stroink, M. (2014). Building effective relationships for community-engaged scholarship in Canadian food studies. Canadian Food Studies, 1(1), 27–53. Retrieved from https://canadianfoodstudies.uwaterloo.ca/index.php/cfs/article/view/19
- Astin, A. W., Vogelgesang, L. J., Ikeda, E. K., & Yee, J. A. (2000). How service learning affects students. Los Angeles, CA: Higher Education Research Institute, University of California.
- Bandy, J., Bartel, A. S., Clayton, P. H., Gale, S., Mack, H., Metzker, J., … & Stanlick, S. (2016). Values-engaged assessment: Reimagining assessment through the lens of democratic engagement. Michigan Journal of Community Service Learning, 23(1), 96–101.
- Berinyuy, C. M., Hallie, E., McDaniel, M., Chapman, D. F., Pendlebury, S., Ford, C. J., & Swap, R. J. (2014, Fall). The adaptive cycle as a lens for service learning: Community engagement partnerships. Partnerships: A Journal of Service-Learning & Civic Engagement, 5(2), 153–177.
- Biggs, R., Westley, F. R., & Carpenter, S. R. (2010). Navigating the back loop: Fostering social innovation and transformation in ecosystem management. Ecology and Society, 15(2), 9.
- Block, P. (2009). Community: The structure of belonging. San Francisco, CA: Berrett-Koehler Publishers.
- Blouin, D. D., & Perry, E. M. (2009). Whom does service learning really serve? Community-based organizations’ perspectives on service learning. Teaching Sociology, 37(2), 120–135.
- Boyer, E. L. (1996). The scholarship of engagement. Journal of Public Service and Outreach,1 (1), 11–20.
- Bringle, R. G., & Hatcher, J. A. (1996). Implementing service learning in higher education. The Journal of Higher Education, 67(2), 221–239.
- Brown, L.D., Bammer, G., Batliwala, S., & Kunreuther, F. (2003). Framing practice-research engagement for democratizing knowledge. Action Research, 1(1), 81-102.
- Burnes, B. (2005). Complexity theories and organizational change. International Journal of Management Reviews, 7(2), 73–90.
- Burnes, B., Hughes, M., & By, R. T. (2018). Reimagining organisational change leadership. Leadership, 14(2), 141–158.
- Bushouse, B. K. (2005). Community nonprofit organizations and service-learning: Resource constraints to building partnerships with universities. Michigan Journal of Community Service Learning, 12(1), 32–40.
- Chazdon, S., Emery, M., Hansen, D., Higgins, L., & Sero, R. (2017). A field guide to ripple effects mapping. Minneapolis, MN: University of Minnesota Libraries Publishing.
- Clayton, P. H., & O’Steen, W. L. (2010). Working with faculty: Designing customized developmental strategies. In B. Jacoby & P. Mutascio (Eds.), Looking in, reaching out: A reflective guide for community service-learning professionals (pp. 95–135). Boston, MA: Campus Compact.
- Davies, R., & Dart, J. (2005). The “most significant change” (MSC) technique: A guide to its use. United Kingdom: CARE International.
- Denizen, N. K. (2009).Qualitative inquiry under fire: Toward a new paradigm dialogue. Walnut Creek, CA: Left Coast Press.
- Dooley, K. J. (1997). A complex adaptive systems model of organization change. Nonlinear Dynamics, Psychology, and Life Sciences, 1(1), 69–97.
- Earl, S., Carden, F., & Smutylo, T. (2001). Outcome mapping: Building learning and reflection into development programs. Ottawa, ON: International Development Research Centre.
- Enos, S., & Morton, K. (2003). Developing a theory and practice of campus-community partnerships. In B. Jacoby & Associates (Eds.), Building partnerships for service-learning (pp. 20–41). San Francisco, CA: Jossey-Bass.
- Forsman, J., Linder, C., Moll, R., Fraser, D., & Andersson, S. (2014). A new approach to modelling student retention through an application of complexity thinking. Studies in Higher Education, 39(1), 68–86.
- Goldstein, J. (2008). Conceptual foundations of complexity science: Development and main constructs. In M. Uhl-Bien and R. Marion (Eds). Complexity Leadership Part 1: Conceptual Foundations. (pp. 17 – 48). Charlotte, NC, USA: Information Age Publishing.
- Greene, J. C. (1994). Qualitative program evaluation: Practice and promise. In N. K. Denzin & Y. S. Lincoln (Eds.), Handbook of qualitative research (pp. 530–544). Thousand Oaks, CA: Sage.
- Guastello, S.J. & Liebovich, L.S. (2009). Introduction to nonlinear dynamics and complexity. In S.J. Guastello, M. Koopmans, & D. Pincus (Eds.), Chaos and Complexity in Psychology: The Theory of Nonlinear Dynamical Systems, (pp. 1-36). Cambridge, MA: Cambridge University Press.
- Guillaume, Y. R. F., Dawson, J. F., Priola, V., Sacramento, C. A., Woods, S. A., Higson, H. E., … & West, M. A. (2014). Managing diversity in organizations: An integrative model and agenda for future research. European Journal of Work and Organizational Psychology, 23(5), 783–802.
- Gunderson, L. H., & Holling, C. S. (2002). Panarchy: Understanding transformations in human and natural systems. St. Louis, MO: Washington University Island Press.
- Hardy, L. J., Hulen, E., Shaw, K., Mundell, L., & Evans, C. (2018). Ripple effect: An evaluation tool for increasing connectedness through community health partnership. Action Research, 16(3), 299–318.
- Harrison, B., Nelson, C. H., & Stroink, M. L. (2013). Being in community: A food security themed approach to public scholarship. Journal of Public Scholarship in Higher Education, 3, 91–110.
- Holland, J. H. (1992). Complex adaptive systems. Daedalus, 121(1), 17–30.
- Holland, J. H. (1998). Emergence: From chaos to order. Cambridge, MA: Perseus Books.
- Holland, J. H. (2006). Studying complex adaptive systems. Journal of Systems Science and Complexity, 19(1), 1–8.
- Holling, C. S. (1973). Resilience and stability of ecological systems. Annual Review of Ecology and Systematics, 4, 1–23. doi:10.1146/annurev.es.04.110173.
- Holling, C. S. (2001). Understanding the complexity of economic, ecological, and social systems. Ecosystems, 4(5), 390–405.
- Hollis, J., Kloos, H., & Van Orden, G.C. (2009). Origins of order in cognitive activity. In S.J. Guastello, M. Koopmans, & D. Pincus (Eds.), Chaos and Complexity in Psychology: The Theory of Nonlinear Dynamical Systems, (pp. 206-241). Cambridge, MA: Cambridge University Press.
- Janke, E. M. (2009). Defining characteristics of partnership identity in faculty-community partnerships. In B. E. Moely, S. H. Billig, & B. A. Holland (Eds.), Creating our identities in service-learning and community engagement (pp. 75–102). Charlotte, NC: Information Age Publishing.
- Kahlke, R., & Taylor, A. (2018). Community service-learning in Canada: One size does not fit all. Engaged Scholar Journal: Community-Engaged Research, Teaching, and Learning, 4(1), 1–18.
- Kania, J., & Kramer, M. (2013). Embracing emergence: How collective impact addresses complexity. Stanford Social Innovation Review. Retrieved from https://ssir.org/articles/entry/social_progress_through_collective_impact.
- Kauffman, S. A. (1993). The origins of order: Self-organization and selection in evolution. New York, NY: Oxford University Press.
- Kauffman, S. (1995). At home in the Universe: The search for laws of self-organization and complexity. New York, NY: Oxford University Press.
- Lincoln, Y. S., & Guba, E. G. (1985). Naturalistic inquiry. Thousand Oaks, CA: Sage.
- Lipshitz, R., Popper, M., & Friedman, V. J. (2002). A multifacet model of organizational learning. Journal of Applied Behavioral Science, 38(1), 78–98.
- Malinsky, E., & Lubelsky, C. (2014). Network evaluation: Cultivating healthy networks for social change. Toronto, ON: Centre for Social Innovation. Retrieved from http://socialinnovation.org/wp-content/uploads/2016/08/NetworkEvaluation_Pocket_english.pdf
- Manson, S. M. (2001). Simplifying complexity: A review of complexity theory. Geoforum, 32(3), 405–414. doi:10.1016/S0016-7185(00)00035-X
- Masterson, V. A., Stedman, R. C., Enqvist, J., Tengö, M., Giusti, M., Wahl, D., & Svedin, U. (2017). The contribution of sense of place to social-ecological systems research: A review and research agenda. Ecology and Society, 22(1), 49. doi:10.5751/ES-08872-220149
- Miller-Young, J., Dean, Y., Rathburn, M., Pettit, J., Underwood, M., Gleeson, J., … & Clayton, P. (2015). Decoding ourselves: An inquiry into faculty learning about reciprocity in service-learning. Michigan Journal of Community Service Learning, 22(1), 32–47.
- Mintzberg, H. (1994). The fall and rise of strategic planning. Harvard Business Review, (January–February), 107–115.
- Mishra, B., & Bhaskar, A. U. (2011). Knowledge management process in two learning organisations. Journal of Knowledge Management, 15(2), 344–359.
- Mitchell, M. (2009). Complexity: A guided tour. Oxford, UK: Oxford University Press.
- Mitleton-Kelly, E. (Ed.). (2003). Complex systems and evolutionary perspectives of organisations: The application of complexity theory to organisations. Amsterdam, Netherlands: Elsevier Science Ltd.
- Moore, M.-L., Olsson, P., Nilsson, W., Rose, L., & Westley, F. R. (2018). Navigating emergence and system reflexivity as key transformative capacities: Experiences from a Global Fellowship program. Ecology and Society, 23(2), 38. doi:10.5751/ES-10166-230238
- Morrison, E., & Wagner, W. (2016). Exploring faculty perspectives on community engaged scholarship: The case for Q methodology. Michigan Journal of Community Service Learning, 23(1), 5–14.
- Munz, E. A., Gatchet, R. D., & Meier, M. R. (2018). Integrating service-learning in the public speaking course. Journal of Communication Pedagogy, 1(1), 115–123.
- Nelson, C. H., & McPherson, D. H. (2003). Cultural diversity in social work practice: Where are we now and what are the challenges in addressing issues of justice and oppression? In W. Shera (Ed.), Emerging perspectives on anti-oppressive practice (pp. 81–100). Toronto, ON: Canadian Scholars Press.
- Nelson, C. H., & McPherson, D. H. (2004). Contextual fluidity: An emerging practice model for helping. Rural Social Work, 9, 199–208.
- Nelson, C. H., Stadey, M., & Lyons, A.-A. (2005). Community-academic research partnership: A key recipe for food security. In A. M. Kirbyson (Ed.), Recipes for success: A celebration of food security work in Canada (pp. 27–31). Winnipeg, MB: Social Planning Council of Winnipeg.
- Nelson, C. H., & Stroink, M. (2010). Benefits to students of service-learning through a food security partnership model. In J. Keshen, B. A. Holland, & B. E. Moely (Eds.), Research for what? Making engaged scholarship matter (pp. 171–199). Advances in Service-Learning Research. Charlotte, NC: Information Age Publishing.
- Nelson, C. H., & Stroink, M. L. (2012). Hubs of knowledge creation: Exploring the potential for knowledge co-generation within the post-secondary academy Association of Commonwealth Universities. Interact, November 3.
- Nelson, C. H., & Stroink, M. L. (2014). Accessibility and viability: A complex adaptive systems approach to a wicked problem for the local food movement. Journal of Agriculture, Food Systems, and Community Development, 4(4), 191–206.
- Nicolis, G., & Prigogine, I. (1977). Self-organization in nonequilibrium systems: From dissipative structures to order through fluctuations. New York, NY: Wiley.
- Nicolis, G., & Prigogine, I. (1989). Exploring complexity: An introduction. New York, NY: W. H. Freeman.
- Olney, C., & Grande, S. (1995). Validation of a scale to measure development of social responsibility. Michigan Journal of Community Service Learning, 2(1), 43–53.
- O’Meara, K., & Niehaus, B. (2009). Service-learning is . . . : How faculty explain their practice. Michigan Journal of Community Service Learning, 16(1), 17–32.
- Osberg, D., Biesta, G., & Cilliers, P. (2008). From representation to emergence: Complexity’s challenge to the epistemology of schooling. Educational Philosophy and Theory, 40(1), 213–227.
- Page, S. E. (2015). What sociologists should know about complexity. Annual Review of Sociology, 41, 21–41. doi:10.1146/annurev-soc-073014-112230
- Patton, M. Q. (2008). Utilization-focused evaluation (4th ed.). Thousand Oaks, CA: Sage.
- Patton, M. Q. (2011). Developmental evaluation: Applying complexity concepts to enhance innovation and use. New York, NY: The Guilford Press.
- Patton, M. Q. (2015). Qualitative research and evaluation methods (4th ed.). Thousand Oaks, CA: Sage.
- Pawson, R., & Tilley, N. (2004). Realist evaluation. Retrieved from http://www.communitymatters.com.au/RE_chapter.pdf
- Piers, C. (2007). Emergence: When a Difference in Degree Becomes a Difference in Kind. In C Piers, J.P. Muller, & J. Brent (Eds). Self-Organizing Complexity in Psychological Systems: First Edition. (pp. 83 – 110). Plymouth, UK: Jason Aronson, Inc.
- Plexus Institute. (n.d.). A complexity science primer: What is complexity science and why should I learn about it? Retrieved from http://www.plexusinstitute.org/wp-content/uploads/2018/01/Primer-on-Complexity.pdf
- Popper, M., & Lipshitz, R. (2000). Organizational learning: Mechanisms, culture, and feasibility. Management Learning, 31(2), 181–196.
- Preskill, H., Gopal, S., Mack, K., & Cook, J. (2014). Evaluating complexity: Propositions for improving practice. Retrieved from www.fsg.org/publications/evaluating-complexity
- Reeb, R. N., Snow-Hill, N. L., Folger, S. F., Steel, A. L., Stayton, L., Hunt, C. A., … & Glendening, Z. (2017). Psycho-Ecological systems model: A systems approach to planning and gauging the community impact of community-engaged scholarship. Michigan Journal of Community Service Learning, 24(1), 6–22.
- Royse, D., Thyer, B. A., & Padgett, D. K. (2016). Program evaluation: An introduction to an evidence-based approach (6th ed.). Boston, MA: Cengage Learning.
- Ruff, K., & Olsen, S. (2016). The next frontier in social impact measurement isn’t measurement at all. Stanford Social Innovation Review. Retrieved from https://ssir.org/issue/fall_2016
- Sandy, M., & Holland, B. A. (2006). Different worlds and common ground: Community partner perspectives on campus-community partnerships. Michigan Journal of Community Service Learning, 13(1), 30–43.
- Seifer, S. D., & Connors, K. (Eds). (2007). Faculty toolkit for service-learning in higher education. Scotts Valley, CA: National Service-Learning Clearinghouse. Retrieved from https://www.vanderbilt.edu/oacs/wp-content/uploads/sites/140/faculty-toolkit-for-service-learning.pdf
- Siemers, C. K., Harrison, B., Clayton, P. H., & Stanley, T. A. (2015). Engaging place as partner. Michigan Journal of Community Service Learning, 22(1), 101–104.
- Smith, M. J. (2010). Handbook of program evaluation for social work and health professionals. Oxford, UK: Oxford University Press.
- Smits, P. A., & Champagne, F. (2008). An assessment of the theoretical underpinnings of practical participatory evaluation. American Journal of Evaluation, 29(4), 427–442.
- Stacey, R. D. (1996). Complexity and creativity in organizations. San Francisco, CA: Berrett-Koehler Publishers.
- Stacey, R. D. (2001). Complex responsive processes in organizations: Learning and knowledge creation. London, UK: Routledge.
- Stoecker, R., Beckman, M., & Min, B. H. (2010). Evaluating the community impact of higher education civic engagement. In H. E. Fitzgerald, C. Burack, & S. D. Seifer (Eds.), Handbook of engaged scholarship: Contemporary landscapes, future directions (Vol. 2., pp. 177–196). East Lansing, MI: Michigan State University Press.
- Stroink, M., & Nelson, C. H. (2009). Aboriginal health learning in the forest and cultivated gardens: Building a nutritious and sustainable food system. Journal of Agromedicine, 14(2), 263–269.
- Stroink, M. L., & Nelson, C. H. (2013). Complexity and food hubs: Five case studies from Northern Ontario. Local Environment: The International Journal of Justice and Sustainability, 18(5), 620–635.
- Tandon, R., Singh, W., Clover, D., & Hall, B. (2016). Knowledge democracy and excellence in engagement. Institute of Development Studies Bulletin, 47(6), 19–35.
- Tremblay, C., Singh, W., & Lepore, W. (2017a). Mutual learning and empowering support: Networks and balance between local and global demands. Global University for Innovation (GUNi). Barcelona, Spain: 6th World Report on Higher Education.
- Tremblay, C., Spilker, R., Nagel, R., Robinson, J. C., & Brown, L. (2017b). Assessing the outcomes of community-university engagement networks in a Canadian context. Engaged Scholar Journal: Community-Engaged Research, Teaching, and Learning, 3(2), 1–21.
- Uhl-Bien, M., Marion, R., & McKelvey, B. (2007). Complexity leadership theory: Shifting leadership from the industrial age to the knowledge era. The Leadership Quarterly, 18(4), 298–318.
- Waldrop, M. M. (1992). Complexity: The emerging science at the edge of order and chaos. New York, NY: Touchstone Book.
- Walker, B., & Salt, D. (2006). Resilience thinking: Sustaining ecosystems and people in a changing world. Washington, DC: Island Press.
- Westley, F., & Antadze, N. (2010). Making a difference: Strategies for scaling social innovation for greater impact. The Innovation Journal: The Public Sector Innovation Journal, 15(2), article 2.
- Westley, F. R., Tjornbo, O., Schultz, L., Olsson, P., Folke, C., Crona, B., & Bodin, Ö. (2013). A theory of transformative agency in linked social-ecological systems. Ecology and Society, 18(3), 27. doi:10.5751/ES-05072-180327
- Westley, F., Zimmerman, B., & Patton, M. Q. (2006). Getting to maybe: How the world is changed. Toronto, ON: Random House of Canada.
- Wickersham, C., Westerberg, C., Jones, K., Cress, M. (2016). Pivot points: Direct measure of the content and process of community-based learning. Teaching Sociology, 44(1), 17-27.
- Wilson-Grau, R. (2015). Outcome harvesting. Better Evaluation. Retrieved from http://betterevaluation.org/plan/approach/outcome_harvesting
- Worrall, L. (2007). Asking the community: A case study of community partner perspectives. Michigan Journal of Community Service Learning, 14(1), 5–17.
- Yang, Y., & Konrad, A. M. (2011). Diversity and organizational innovation: The role of employee involvement. Journal of Organizational Behavior, 32(8), 1062–1083.
- Zimmerman, E. B., Haley, A., Creighton, G. C., Bea, C., Miles, C., Robles, A., … & Aroche, A. (2019, Winter). Assessing the impacts and ripple effects of a community-university partnership: A retrospective roadmap. Michigan Journal of Community Service Learning, 25(1), 62–76.
Authors
DR. CONNIE NELSON ([email protected]) is a Professor Emeritus at Lakehead University in Ontario. She is a specialist in community capacity building, regional food system development, and organizational development.
DR. MIRELLA STROINK ([email protected]) is an Associate Professor and Chair of the Department of Psychology at Lakehead University in Ontario. Her research is rooted in the application of complex adaptive systems theory to understanding human cognition and behavior in the ecological and social context.
Drs. Stroink and Nelson are co-founders of the 180 Institute, which approaches complex challenges of society through the lens of complexity science. http://www.180institute.com/