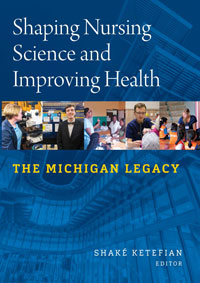
Shaping Nursing Science and Improving Health: The Michigan Legacy
Skip other details (including permanent urls, DOI, citation information) :This work is licensed under a Creative Commons Attribution-NonCommercial-NoDerivatives 3.0 License. Please contact : [email protected] to use this work in a way not covered by the license.
For more information, read Michigan Publishing's access and usage policy.
Short Paper: Health Science Analytics: Data- and Technology-Driven Approaches for Addressing Health Care Challenges, Advancing Well-Being, and Enhancing Nursing Education
There are many health care challenges that currently inhibit our ability to respond quickly, effectively, and decisively in addressing acute and chronic, urgent and ambulatory, and short- and long-term care. At the same time, there are enormous opportunities for change, improvement in health practice and scientific advances that are driven by rapid scientific progress, national health care policies, accelerated IT developments, and tremendous training and curricular improvements.
Challenges
Globally, there is a significant push to improve short-term and long-term self-management care; reduce individual, institutional or government costs associated with a multitude of acute and chronic conditions; and develop effective evidence-based decision-support systems. The Patient Protection and Affordable Care Act (2010) promotes health care coverage for low- and middle-income families and businesses; however, it may be years before its impact on individuals and the entire health system is fully understood (e.g., compliance, benefits, costs, detriments, potential to advance our knowledge, and ability to deliver and track care). Addressing these challenges requires a broad range of expertise, a commitment to transdisciplinary science, the development of new training curricula, continued scientific advances, reliable, and scalable infrastructure for data management and analytics. Other critical factors include efficient and sustained funding support and effective regulatory and policy frameworks that maximize returns on investment, protect personal information, and secure sensitive materials (e.g., biohazards) (Toga & Dinov, 2015). There are also substantial IT challenges that demand considerable attention and tangible progress. For instance, transitioning to the 10th revision of the World Health Organization’s International Statistical Classification of Diseases and Related Health Problems (ICD-10; http://www.CMS.gov/Medicare/Coding/icd10) required care providers to allocate time, resources, and staff for its effective implementation and emersion in clinical practice to meet the regulatory October 2015 deadline. Figure 10-a shows the new alphanumeric labeling nomenclature for annotating and cataloging human health conditions, diseases, symptoms, abnormal findings, and injuries.
Big Data Science
The following are some of the very distinctive characteristics that make big data unique and very different from traditional (small) data: (a) size—the volume of data often exceeds standard storage, memory and, computational capabilities; (b) incongruency—big data samples, cases, and observations may be highly nonhomologous, which requires special treatment; (c) incompleteness—big data might be sparse, with (random and nonrandom causes of) missing values due to the en masse nature of its generation and assembly; (d) complexity—as the dimension of the data increases, distance metrics between observations become degenerate (curse of dimensionality), leading to considerable computational and interpretation challenges, (e) multiscale—big data frequently includes observations from micro to macro scales spanning time, space, and frequency spectra; and (f) multisource—numerous digital, analogous, and mixed data are produced by devices and instruments, which demand special protocols for the integration (fusion) of relevant data within and beyond the scope of any specific research study. The model, algorithm, and tool development necessary to cope with these specific challenges make “standard scientific methods” impractical for understanding big data. Cleveland (2001) introduced the notion of data science as an independent discipline, extending the field of statistics to incorporate six technical areas: multidisciplinary investigations, models and methods for data, computing with data, pedagogy, tool evaluation, and theory. Data science is an applied scientific field crossing many discipline boundaries to derive valuable insights and actionable knowledge from complex observable phenomena. Data science practitioners require a versatile and unique set of skills to manage, process, interrogate, and extract information from complex systems.
Opportunities
Significant health care opportunities derive from three complementary sources: ubiquitous big health care datasets, enormous methodological and technological advances, and the emergence of data science analytics. Figure 10-b shows two examples of the very rapid increase of the volume and complexity of data acquired to support a wide spectrum of biomedical, clinical, and health care needs. This data avalanche presents generous opportunities to examine, model, treat, and track normal and pathological conditions (Dinov et al., 2014).
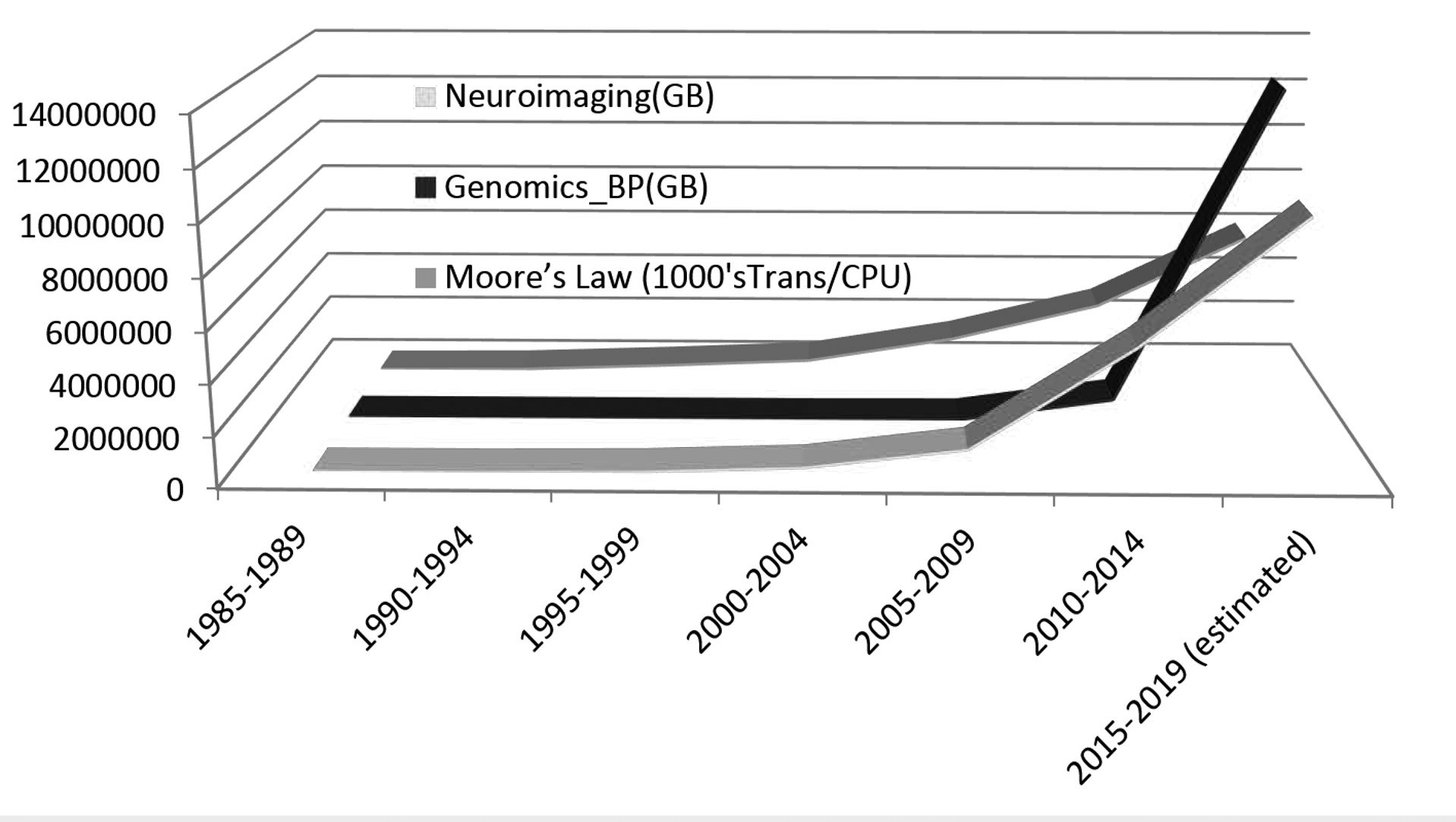
Exponential growth of health care data illustrated using neuroimaging and genomics. The misalignment between the rapid rate of increase of the volume of data and the increase of computational power necessary for the information processing is the result of enormous technological advances and improvements in data resolution, streaming efficiency, and censoring equipment. By 2015, more than 106 whole human genomes will be sequenced, totaling more than 100 petabytes of data.
The impressive methodological and technological advances in health care introduced since 2010 include scientific discoveries, methodological improvements, and technological products that collectively alleviate suffering, cure diseases, improve quality of life, and provide the foundation for bigger breakthroughs in the near future. Examples of such innovative developments include smart clinical trials; protocols for deep understanding of the phenology, genetics, and environmental effects on neurodegeneration (e.g., Alzheimer’s and Parkinson’s); rapid and effective blood tests using microsamples; high-frequency wireless wearable technologies (Figure 10-c), and powerful data analytic approaches (work by Dinov under review).
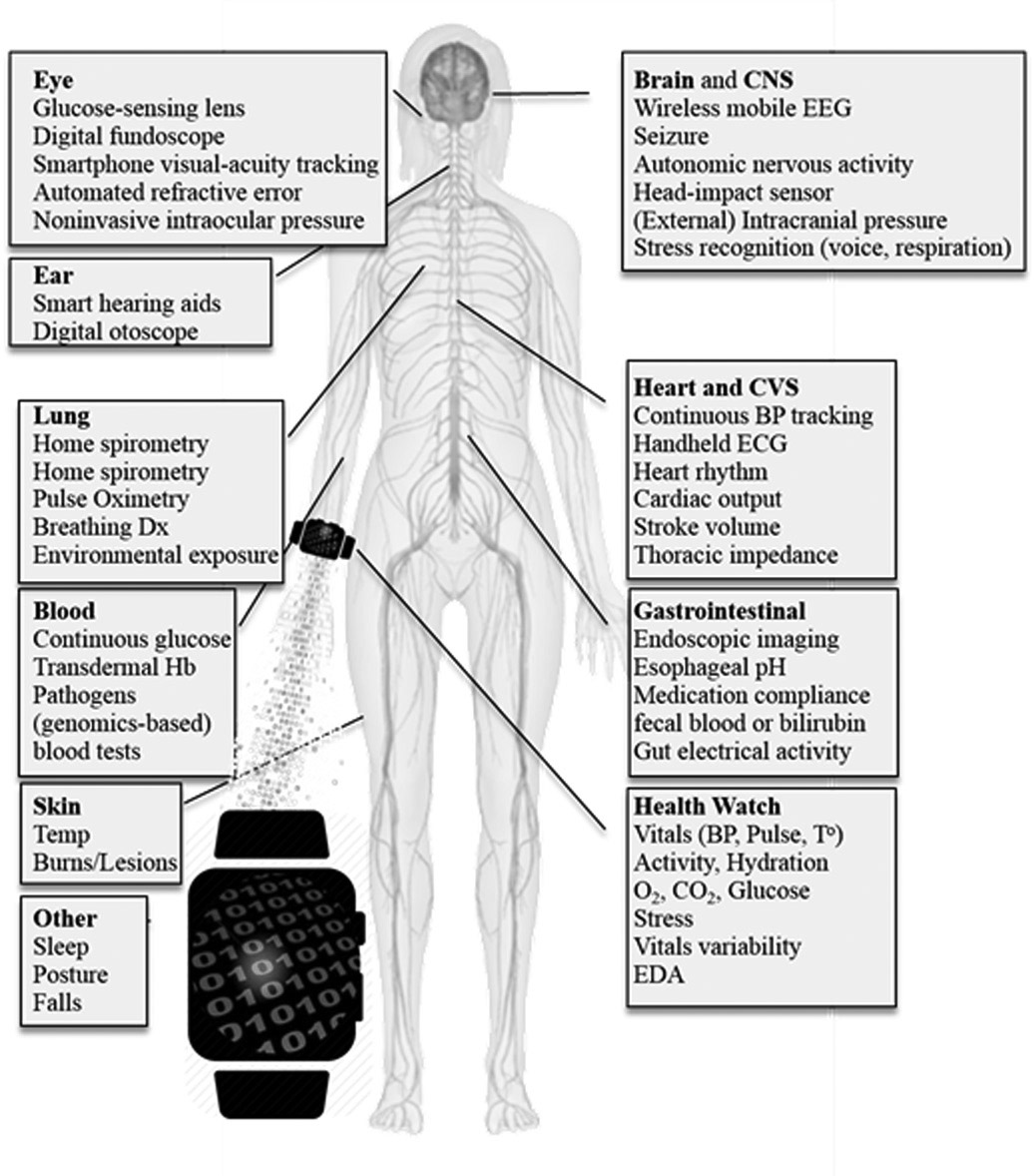
>The innovative health care advances since 2010 include continuous monitoring using wearable technologies. This provides big health care data associated with location, time, and metabolic and biological characteristics that can be harvested, streamed, modeled, analyzed, visualized, and interpreted in real time.
The emergence of data science analytics coincides with a wave of innovative scientific discoveries that enable predictive modeling and high-throughput analytics that are critical for interrogating big health care data and gaining insights about patterns, trends, connections, and associations in the data. The unique characteristics of such datasets trade off the importance of traditional hypothesis-driven inference and statistical-significance with computational-efficiency, protocol-complexity, and methods-validity (Dinov, Siegrist, Pearl, Kalinin, & Christou, 2015; Husain, Kalinin, Truong, & Dinov, 2015).
Nursing and Health Science Research and Education
Effective management and efficient health care delivery depend on rapid evidence-based, big-data driven, vibrant, robust decision making. Effective management requires unique skill sets; broad knowledge; hands-on experience; teamwork; and the successful integration of human knowledge, machine intelligence, and powerful hardware resources. Many communities and organizations involved in nursing and health research, training, funding, and practice advocate for a significant overhaul in health science training. This includes approaches, techniques, and the implementation of basic and advanced statistical and analytical methods in the undergraduate and graduate health science curriculum, at the PhD level (Wyman & Henly, 2015), and at earlier levels of education as well. Prior recommendations (Bednash, Breslin, Kirschling, & Rosseter, 2014) include enhancing the links between rigorous education and effective practice, modernization of the curriculum (e.g., advanced methods, IT integration, transdisciplinary training), ongoing valuation of training effectiveness, apprentice programs and partnerships for innovative data-driven discoveries, placements in appropriate clinical residency programs, active methodological learning, the blending of domain-specific knowledge, clinical abilities and data analytic skills, and pairing rigorous scientific training with clinical reasoning and quantitative literacy. For instance, the report The Research-Focused Doctoral Program in Nursing: Pathways to Excellence (American Association of Colleges of Nursing, 2010) recommends that PhD nursing students be exposed to formal and informal learning experiences; build scientific depth in an identified area of study; learn advanced research design and statistical methods; and develop skills for data, information, and knowledge management, efficient processing, and hands-on analysis.
The adoption of innovative scientific methods in advanced nursing education, health care research, and clinical practice could be improved. Many factors may inhibit the adoption of advanced analytical methods into the training curriculum, for example, care demands on practicing health care providers, demographics of learners and instructors, the DNP/PhD dichotomy, and the powerful inertia of the status quo (Smeltzer et al., 2015). There may not be one unique solution for improving the analytical and scientific skills of nursing professionals. We should enhance the quality and increase the robustness of nursing research while we simultaneously refine the baccalaureate, master’s, and doctoral programs. The community needs to review the broad spectrum of modern scientific methods for health sciences and identify key statistical and analytic concepts critical for students’ growth as skilled health care professionals, scholars, and practitioners. Some statistics techniques are already an integral part of nursing practice and research.
The faculty of the University of Michigan School of Nursing designed and implemented a novel core statistical and analytics training program for nursing professionals that includes a blend of courses emphasizing the theoretical foundations, model assumptions, computational tools, and applied research practice involving contemporary qualitative and quantitative methods. Table 10-a illustrates this series of four graduate courses (4 credits each) that provide the foundation for a new graduate-level nursing methods and analytics curriculum.
Foundation courses | Advanced courses | ||
---|---|---|---|
Fundamentals | Applied Inference | Linear Modeling | Special Topics |
Objectives | Objectives | Objectives | Objectives |
Apply data management strategies to sample data files | Understand the commonly used statistical methods of published scientific papers | Compare and contrast advanced statistical concepts, grasp model assumptions/limitations, and apply them to quantitative analyses in health care research | Research, employ, and report on recent advanced health sciences analytical methods |
Carry out statistical tests to answer common health care research questions using appropriate methods and software tools | Conduct statistical calculations/analyses on available data | Apply multivariate statistical modeling, enabling consistency between research questions and selected advanced statistical analyses | Read, comprehend, and present recent reports of innovative scientific methods applicable to a broad range of health problems |
Understand the core analytical data-modeling techniques and their appropriate uses | Use software tools to analyze specific case-study data | Critique and select appropriate advanced statistical linear models. | Experiment with real Big Data |
Communicate advanced statistical concepts/techniques | Conduct multivariate statistical analyses | ||
Determine, explain, and interpret assumptions and limitations | Conduct multivariate statistical analyses |
Foundation courses | Advanced courses | ||
---|---|---|---|
Fundamentals | Applied Inference | Linear Modeling | Special Topics |
Topics | Topics | Topics | Topics |
Exploratory data analytics | Epidemiology | Multiple regression | Scientific visualization |
Parametric inference | Correlation/regression | General linear model | PCOR/CER methods |
Probability theory | ρ and slope inference, 1–2 samples | ANOVA | HTE |
Odds ratio/relative risk | ROC curve | ANCOVA | Big Data / Big Science |
Distributions | ANOVA | MANOVA | Big Data / Big Science |
Exploratory data analysis | Nonparametric inference | MANCOVA | Missing data |
Resampling/simulation | Cronbach’s α | Repeated measures ANOVA | GWAS |
Design of experiments | Measurement reliability/validity | Time-series analysis | Medical imaging |
Intro to epidemiology | Survival analysis | Fixed, randomized, and mixed models | Data networks |
Estimation | Decision theory | Hierarchical linear models | Adaptive clinical trials |
Hypothesis testing | Central limit theorem | Mixture modeling | Databases/registries |
Experiments vs. observational studies | Association tests | Surveys | Meta-analyses |
Data management | Bayesian inference | Longitudinal data | Causality/causal inferences |
Power, sample-size, effect-size, sensitivity, and specificity | PCA/ICA/factor analysis | Generalized estimating equations (GEE) models | SEM |
Association vs. causality | Point/interval estimation (CI) | Model fitting and model quality (KS-test) | Classification methods |
Clinical vs. statistical significance | Study/research critiques | Time-series analysis | |
Statistical independence Bayesian rule | Common misconceptions | GIS | |
Rasch measurement model | |||
MCMC Bayesian inference | |||
Network analysis |
The value of this new nursing/health science curricular redesign is threefold. First, it will build the trainees’ core skills for dealing with an avalanche of health care data, which will promote swift data-driven decision making, smart reactions, and competent responses to varying health care observations. Second, it will enable and galvanize transdisciplinary collaborations among basic scientists, clinical investigators, and health care practitioners to solve complex biomedical problems. Third, the new curriculum aims to enhance the processes of patient diagnosis, treatment, prevention of human disease or injury, and management of other physical and mental impairments. These benefits might be realized by utilizing modern scientific techniques, embedding data-driven inference in the decision-making discoveries, and avoiding common mistakes in various health care settings.
Past, Present, and Future
Much of the foundation of modern health and nursing science is deeply rooted in the development and utilization of innovative scientific methods for data modeling, statistical analysis, and evidence-based practice. For instance, Florence Nightingale (1820–1910), the founder of modern nursing science, established the first professional nursing school at St. Thomas’ Hospital in London (King’s College London). She recognized early on the importance of broad-based scientific training, including mathematics and statistics, to aggregate, analyze, and demonstrate evidence-based health care practice. Nightingale was a pioneer in the graphical presentation of information and developed the widely used polar area plot for radial display of frequency patterns, which she used to depict the observed cyclical trends of soldier mortality (Figure 10-d). More of these core data analytic contributions, statistical methodological developments and fundamental scientific discoveries are necessary to attract and train skilled nursing and health care scientists, advance the biomedical and health care research, bridge across transdisciplinary boundaries, and ultimately improve human health.
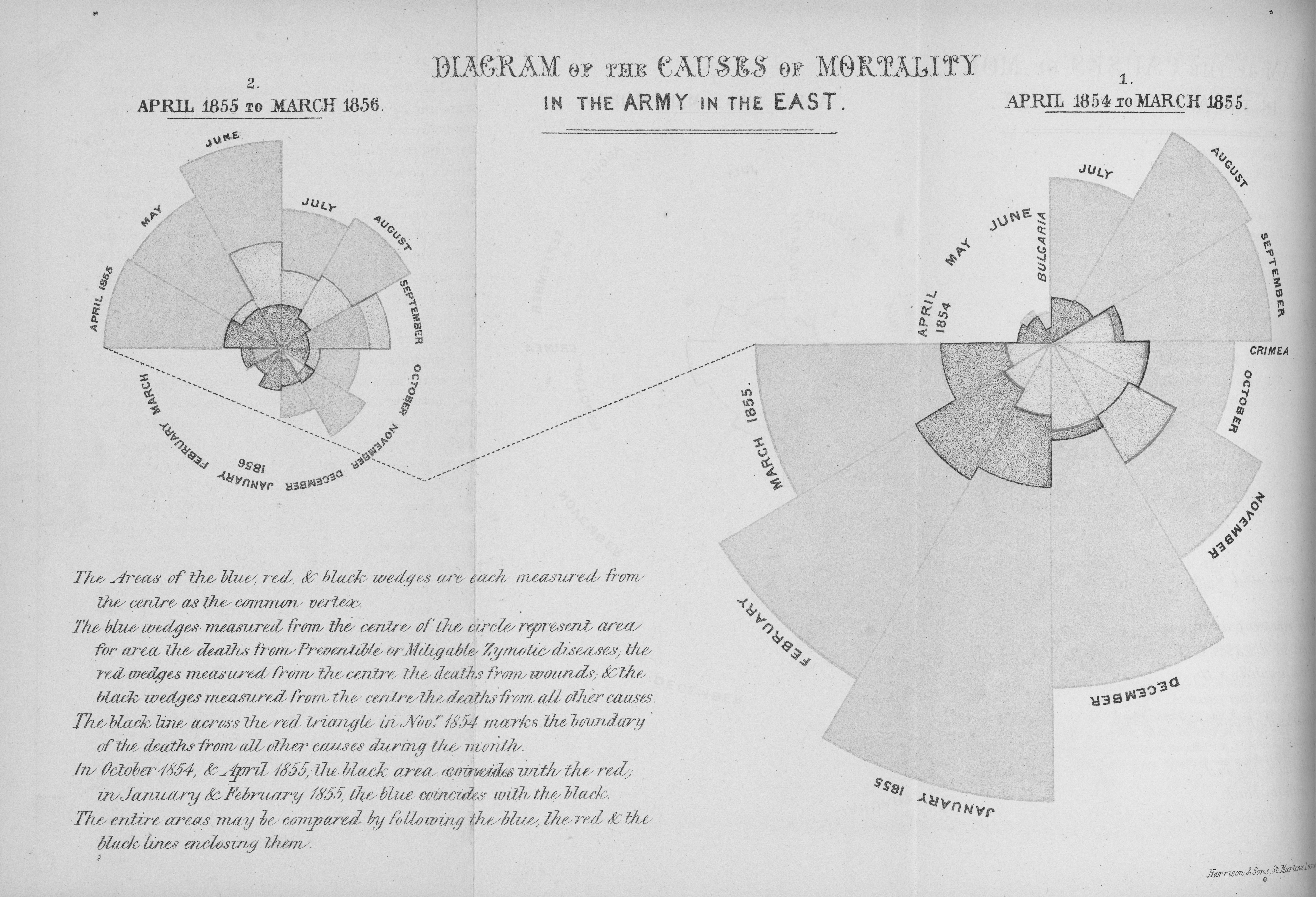
Nursing scientific innovation—Nightingale’s polar area plot showing observed cyclical trends of soldier mortality.
In the past year, we have developed a mechanism to integrate dispersed multisource data; service the mashed information via human and machine interfaces in a secure, scalable manner; and enable joint data analytics (Husain et al., 2015). This new platform includes a device agnostic tool (Dashboard web app: http://socr.umich.edu/HTML5/Dashboard) for graphical querying and navigating and exploring the multivariate associations in complex heterogeneous datasets (Figure 10-e).
References
- American Association of Colleges of Nursing. (2010). The research-focused doctoral program in nursing: Pathways to excellence. Washington, DC: Author.
- Bednash, G., Breslin, E. T., Kirschling, J. M., & Rosseter, R. J. (2014). PhD or DNP planning for doctoral nursing education. Nursing Science Quarterly, 27(4), 296–301.
- Cleveland, W. S. (2001). Data science: An action plan for expanding the technical areas of the field of statistics. International Statistical Review, 69(1), 21–26. doi:10.1111/j.1751-5823.2001.tb00477.x
- Dinov, I. D., Petrosyan, P., Liu, Z., Eggert, P., Zamanyan, A., Torri, F., . . . Toga, A. W. (2014). The perfect neuroimaging-genetics-computation storm: Collision of petabytes of data, millions of hardware devices and thousands of software tools. Brain Imaging and Behavior, 8(2), 311–322.
- Dinov, I. D., Siegrist, K., Pearl, D. K., Kalinin, A., & Christou, N. (2015). Probability distributome: A web computational infrastructure for exploring the properties, interrelations, and applications of probability distributions. Computational Statistics, 594, 1–19. doi:10.1007/s00180-015-0594-6
- Husain, S. S., Kalinin, A., Truong, A., & Dinov, I. D. (2015). SOCR Data dashboard: An integrated big data archive mashing Medicare, labor, census and econometric information. Journal of Big Data, 2(13), 1–18. doi:10.1186/s40537-015-0018-z
- Patient Protection and Affordable Care Act, 42 U.S.C. § 18001 (2010).
- Smeltzer, S. C., Sharts-Hopko, N. C., Cantrell, M. A., Heverly, M. A., Nthenge, S., & Jenkinson, A. (2015). A profile of U.S. nursing faculty in research- and practice-focused doctoral education. Journal of Nursing Scholarship, 47(2), 178–185.
- Toga, A. W., & Dinov, I. D. (2015). Sharing big biomedical data. Journal of Big Data, 2(1), 7.
- Wyman, J. F., & Henly, S. J. (2015). PhD programs in nursing in the United States: Visibility of AACN core curricular elements and emerging areas of science. Nursing Outlook, 63(4), 390–397.