Socio-economic Drivers of Community Garden Location and Quality in Urban Settings and Potential Effects on Native Pollinators
Skip other details (including permanent urls, DOI, citation information)
:
This work is licensed under a Creative Commons Attribution-NonCommercial-NoDerivatives 3.0 License. Please contact : [email protected] to use this work in a way not covered by the license.
For more information, read Michigan Publishing's access and usage policy.
Abstract
Concurrent with increased urban agriculture in American cities over the past decade, questions have arisen concerning how gardens spread and what effects they have on local ecosystems. Socio-economic demographics may factor into patterns of garden placement and quality, which lead to changes that could affect biotic communities in ways urban ecologists are only beginning to understand. Some changes are potentially beneficial. For example, researchers hypothesize that increased floral resources in gardens could benefit declining pollinator species. However, the types and extent of such connections between socio-economic drivers and ecological effects are poorly understood. Here we present a case study of urban community gardens in southeast Michigan and potential effects on floral resources and native pollinator communities. We created socio-economic profiles of communities surrounding gardens using census land cover data to discern patterns in garden placement. A subset of these gardens was then sampled for floral resources and bees. In general, results show that gardens are evenly dispersed across communities with varying socio-economic characteristics, indicating that other factors, such as land availability, may be greater determinants of garden locations. However, socio-economic variables significantly correlate with garden floral qualities, which may have implications for urban pollinator support. The findings of our study have relevant policy implications: cities looking to reap the environmental benefits of gardens should consider creating policies and initiatives that support and enable the development of community gardens. Overall we present a novel combination of techniques to connect social and ecological components of sustainability.
Introduction
In recent years, urban agriculture has seen a marked increase in prevalence across the United States. According to estimates by the National Gardening Association, the number of urban gardeners increased by 29 percent between 2008 and 2013, and the number of households participating in community gardening increased by 200 percent to over three million during the same time period (National Gardening Association 2014). Such trends give rise to questions about how and why gardens spread, as well as where they get established and who stands to benefit most. Urban and community gardens have been touted for their ability to address a wide range of issues including food security, urban blight, community cohesion, public health, poverty and unemployment, and environmental sustainability (Santo et al. 2016). The purported sustainability potential of urban agriculture has been attributed to reduction of food miles and greenhouse gas emissions (Kulak et al. 2013), sequestration of air pollutants into plant tissue (Janhäll 2015), biodiversity preservation (Galluzzi et al. 2010), and other ecosystem services. Yet empirical impacts vary greatly with context and require more research (Santo et al. 2016).
Studies have also examined what effects gardens have on surrounding communities using a variety of indicators, and socio-economic effects of gardens are a common area of inquiry. For example, a 2012 review of urban community garden literature examined 11 US studies that measured the effect of gardens on surrounding property values, all of which found an increase (Guitart et al. 2012). Yet most academic studies thus far have not addressed whether particular types of communities are more prone to establish and maintain community gardens than others. In other words, it is prudent to study not only how socio-economic variables are affected by community gardens, but also how they may be determinants of community garden persistence and quality.
Socio-demographic differences across communities might affect the underlying environmental quality and resultant ecological structure of gardens. This might be especially true in decentralized community gardens managed by individual residents rather than institutions. Links between socio-economic characteristics and the biodiversity of plants (Grove et al. 2006, Kinzig et al. 2005; Hope et al. 2003) and birds (Loss et al. 2009; Kinzig et al. 2005) in US cities have been studied at a variety of scales ranging from home gardens to neighborhoods and landscapes. Plant biodiversity in itself has been demonstrated as an important determinant of the status of animal and insect communities (Knopps et al. 1999), and this might be particularly true in urban gardens (Smith et al. 2006). For example, it has been suggested that the floral resources in urban gardens might be a valuable resource for at-risk bee communities (Hernandez et al. 2009), which are suffering global declines (IPBES 2016). Yet the broader connection between socio-economic variables and the ability of gardens to support bees remains understudied.
Bees provide an indispensable service to ecosystems by pollinating plant communities so as to maintain their genetic diversity and resilience. Lack of animal pollinators is the most common proximate cause of reproductive impairment in wild flowering plants, 80 percent of which depend directly on insects for this purpose (Potts et al. 2010). Bees are also responsible for the increased quantity, quality, and stability of over 60 percent of the world’s crops (Garibaldi et al. 2011). This agricultural service is appraised at nearly $200 billion annually (Gallai 2009). Beyond domesticated honeybees, wild bees are of particular interest because of their highly significant positive impact on yields. A study of 41 crop systems worldwide, found that “fruit set increased significantly with wild insect visitation in all crop systems, but with honey bee visitation in only 14 percent of the systems,” and that “fruit set increased twice as strongly with visitation by wild insects as with visitation by honey bees” (Garibaldi et al. 2013).
One of the largest probable causes of global bee declines is habitat loss and fragmentation, driven in part by increasing urbanization (Goulson et al. 2015). Meta-analyses have found significant negative effect of distance from natural habitat on the richness and abundance of wild bees in particular (Potts et al. 2010). Community gardens might be considered an attractive and straightforward solution to this problem—in theory, planting more flowers should offer bees more resources in urban centers and act as corridors between fragmented natural habitats. Indeed, some studies provide evidence that this may be the case (Pardee and Philpott 2014; Potter and LeBuhn 2015), while others have found floral additions do not necessarily enhance bee abundance or richness in urban gardens (Matteson 2010). Considering aspects of garden quality besides general flower abundance may be necessary to explain the potential of urban gardens to sustain healthy urban pollinator communities. Yet this raises the question of what drives garden floral quality. Beyond ecological conditions, characteristics related to who uses the garden could be influencing factors. Assessing how socio-economic demographics affect the prevalence of gardens across different communities and their floral compositions may be important to understand the habitat connectivity and resources availability for pollinators in cities. Such understanding would be invaluable for informing desperately needed policy initiatives at local, state, and federal levels to maximize benefits to both human and pollinator communities (Inouye et al. 2017).
Here we present a case study of community gardens in Ann Arbor and Ypsilanti, Michigan to investigate the relationships among socio-economic demographics, community garden placement, garden floral quality, and bee community composition. Given the inherently interdisciplinary nature of this work, we employ a diverse set of methods spanning the natural and social sciences to analyze complex socio-ecological trends. Specifically, we ask three questions:
- Are community gardens more prevalent in certain socio-economic conditions of urban environments? Specifically, do socio-economic variables correlate with garden placement in Ann Arbor and Ypsilanti, Michigan?
- How do socio-economic conditions of the surrounding community correlate with community garden floral resource quality?
- How does floral resource quality influence the pollinator abundance in urban community gardens?
Methods
Study Sites
Our study encompasses 30 community gardens maintained by management organizations Project Grow in Ann Arbor and Growing Hope in Ypsilanti. These particular sites are advantageous for addressing our research questions. Both management organizations maintain readily available data about community garden locations and administer consistent management regulations across garden sites. Furthermore, the Ann Arbor/Ypsilanti area encompasses significant heterogeneity of socio-economic demographics within a limited geographic range, both making our study logistically feasible and limiting the effect of confounding variables such as divergent climates.
During the summer of 2014 we received permission to sample bee communities and floral resources across a subset of 11 of the original 30 garden sites. These gardens were located at Buhr Park, Clague Middle School, the University of Michigan Campus Farm, County Farm Park, Catholic Social Services, Eastern Michigan University, Frog Island, Greenview, Normal Park, Perry Community Garden, and West Park. All gardens ban the use of neonicotinoid pesticides—which have been linked to declining bee health (Woodcock et al. 2016; Blacquière et al. 2012)—and provide guidelines to utilize organic growing practices accepted by major organic certification agencies, such as the Organic Crop Improvement Association (http://www.ocia.org/).
GIS and socio-economic Data
We used socio-economic/demographic indicators related to wealth from the US Census Bureau’s 2006-2010 American Community Survey in our analyses. These included median income, percent below the poverty line, population percent with a bachelor’s degree, median property value, and median age. All of the socio-economic variables considered here are associated with wealth in Ann Arbor and Ypsilanti. Median income has significant positive correlations with age, percent bachelor’s degree, and property value, and a negative correlation with poverty rate. These associations correspond to our understanding of Ann Arbor/Ypsilanti demographics. Additionally, both cities are college towns, and official census rules dictate that most college students should be counted at their college addresses (census.gov/2010census).
Profiles for each site regarding each socio-economic variable were created using Geographic Information System (GIS) programs. Garden locations were aggregated with available data from Project Grow and Growing Hope. GIS was used to create quarter-mile buffer zones around each of 30 garden sites in Ann Arbor and Ypsilanti and overlay these buffers with census tract data for each socio-economic variable. We determined the proportion of each census tract that lay within a particular buffer, multiplied the variables by this proportion, and summed the proportional values to attain the socio-economic data values associated with each garden site.
To analyze the relationship between garden location and level of urban development, we used the proxy variable impervious surfaces. Impervious surfaces are roads, buildings, parking structures, or anything else that effectively blankets the surface with concrete or building material. To calculate the amount of impervious surface around each site, National Land Cover Database data from 2011 (Multi-Resolution Land Characteristics Consortium, mrlc.gov) was used. In keeping with literature suggestions (McKinney 2008) of defining urban landscapes as areas with >50 percent impervious surface, areas categorized as high (80-100 percent impervious) and medium (50-79 percent impervious) density developed were summed to obtain the total area of impervious surface within 500-meter buffer zones around each individual sampling site. Dividing that total area of impervious surface by the overall land area resulted in the percent impervious surface for each buffer zone of each sampling site.
Field Sampling
Insect trapping methods for this project are consistent with those commonly found in the literature (Pardee and Philpott 2014; Fortel et al. 2012; Matteson 2007). Methods and frequency of sampling used for this study adhered with recommendations to avoid disrupting bee communities while maintaining accurate representation in data (Banaszak et al. 2014).
Pan trapping was performed every two weeks from May to September 2014. At each site, pan traps were installed in a rectangular arrangement eight square meters. Pan traps were coated with UV reflecting paint to attract insects that forage using UV light as a guide; two of each color blue, yellow, and white were placed at each site to account for species’ varying preferences for particular color wavelengths. Pan traps were filled with water mixed with a small amount of Dawn scentless soap, which acts to reduce the surface tension of the water and thereby increases the likelihood that insects will sink and be trapped effectively. At sites unobscured by floral cover, the trap apparatus is left at ground level, whereas it is mounted on PVC pipes at sites where the ground is covered by vegetation.
In addition to passive trapping, active netting was done every other collection date (once per month) so as to ensure sampling of larger species whose size or flying strength make them difficult to capture in pans. For each date on which netting occurred, it was done for 30 minutes both in the morning (defined as 9 a.m. to 12 noon) and in the afternoon (defined as 1 p.m. to 4 p.m.) within a 5-meter square centered on the trap apparatus. Trapping was done twice per day to account for temporal variation among various bee communities.
To measure the amount of floral resources available to pollinators per site, floral surveys were conducted on each trap date. As measuring and counting every single flower was not feasible, a logarithmic scale was used to estimate the number of flowers of each species in a 20-meter radius circle around the center of the trap setup at each garden. Species average measurements of floral geometry were used along with count estimates to calculate the geometric area of open inflorescence (hereafter referred to as “floral area”) for individual species and total flowers present within the 1256.64 meters square sampling area.
We evaluated garden floral quality based on mean floral area and diversity. Floral area and diversity were averaged across sampling dates per sites to account for variance in floral resources due to harvesting and sampling availability. We also evaluated specific subdivisions within floral community composition. These included the mean and proportional areas of crop, herb, ornamental, weed, and weed/ornamental plant species, as well as introduced, native, introduced/native, and invasive plant species. Species categorizations were determined based on literature review.
Statistical Analyses
Data was compiled in GIS and statistical analysis was completed using the statistical software R. In order to address question 1 of this study, socio-economic and demographic variables at garden locations were compared to areas without gardens. To accomplish this, buffer zones were created for randomly chosen non-garden locations. Then socio-economic variables and impervious surface coverage were measured within the buffer zones similarly to garden locations (see GIS section). Using non-garden locations as reference points is more applicable than simply comparing garden locations to overall city averages. Using city averages would result in a fallacious comparison: garden locations would be tested against a broader landscape also containing those same garden locations. In other words, we would be comparing something to itself.
Socio-economic variables at garden locations and non-garden locations were compared directly through t-tests. Additionally, the effect of physical urban development on garden location was investigated by considering the range of impervious surface coverage around garden and non-garden locations. The amount of impervious surface coverage around each land type was input into a logistic regression which output the probability of finding urban gardens given the amount of impervious surface coverage at any given point in the city.
In addressing question 2, we initially set out to use socio-economic variables as predictors and floral measurements as dependent variables in multivariate regression analysis. In this process we confronted the fact that the socio-economic variables in our data exhibit “multicollinearity.” In other words, the income around gardens strongly and positively correlates with property value, median age, and bachelor’s degree percentage. Conversely, these variables all strongly, negatively correlate with percent in poverty (see Appendix 1.2). Perhaps unsurprisingly, socio-economic variables are very often collinear (Wagner 1982). Multicollinearity can lead to particularly imprecise estimates of beta coefficients in ordinary multivariate regressions. Since tests showed our data exhibits a high degree of multicollinearity (see Appendix 1.2), ordinary multivariate models had to be avoided. We proceeded with the analysis in two ways.
First, analysis of the effects of socio-economic factors on floral quality and subsequent effects on bee abundance was handled using single-variable linear models. Estimates of the beta coefficients of these more straightforward single–variable linear models were verified through residualization in multivariate models. The results presented in this work stem from this analysis, as they are more widely accessible to readers with varying degrees of statistical exposure. Given the use of linear models, skewed variables were log transformed to meet conditions of normality (see Appendix 1.1 for more detail). Data was also standardized to z-scores given that each variable was measured across significantly different ranges and scales, streamlining comparison of effect size across variables.
Second, to more directly address multivariate models and to corroborate coefficient estimates garnered from single variate models, we utilized partial linear square regressions (PLS regressions) to create multivariate regressions. PLS regression is often used when predictor variables are highly collinear (Mevik and Wehrens 2007). In our analysis, PLS regressions acted as a degree of authentication of the relationships found between socio-economic variables and dependent variables (see Appendix 1.2 for further details).
Results
Garden Location
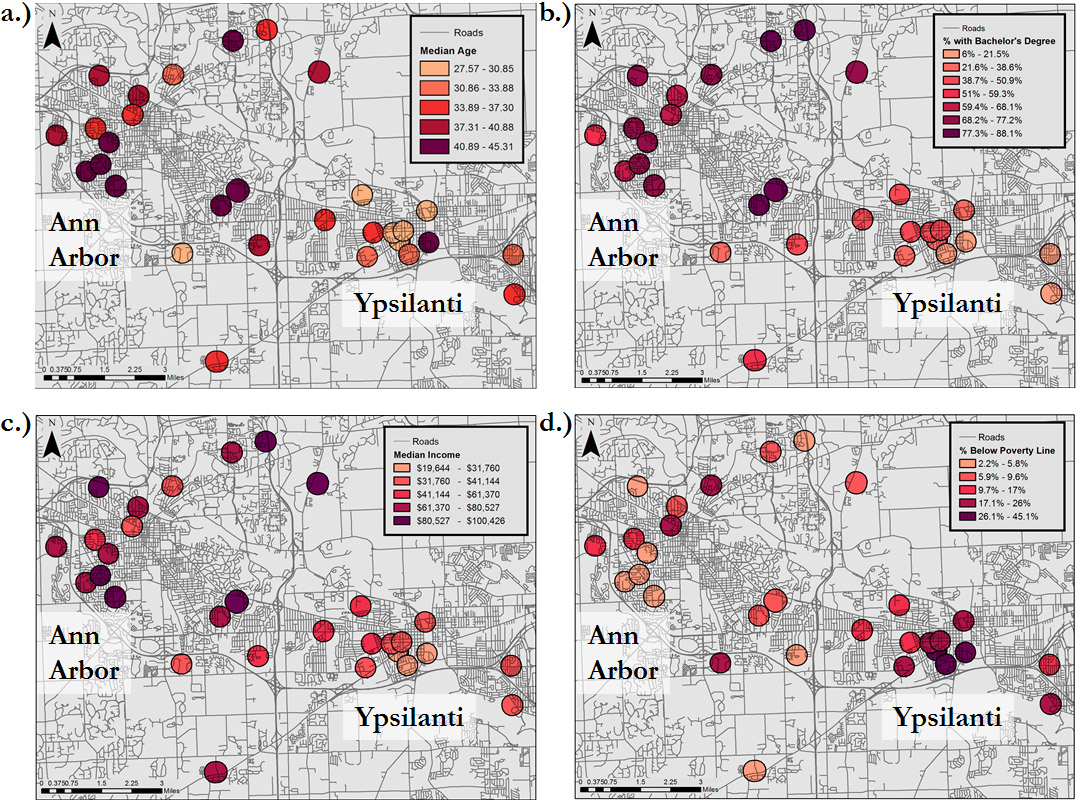
Analysis of the geographic and socio-economic patterns of communities surrounding gardens reveals a number of important quantitative and qualitative observations. Median income and percentage of Bachelor’s degrees in garden buffer areas showed no significant differences from parts of the respective city not containing gardens. In other words, the income and education demographics of neighborhoods surrounding gardens in Ypsilanti tended to reflect those of Ypsilanti as a whole, and the demographics of neighborhoods surrounding gardens in Ann Arbor was similar to Ann Arbor as a whole. Ypsilanti also showed no difference between garden and non-garden areas for the variables of poverty and age. In Ann Arbor, however, areas surrounding community gardens were significantly older (t=-2.766, df=42.344, p=0.008) and had a significantly lower poverty rate (t=2.603, df=51.111, p=0.012) than non-garden areas. These differences might be explained by the pattern of student housing in the city, which will be explicated in the discussion. Additionally, there were significant differences in garden demographics between cities, apparent in the differential shading on the maps in Figure 1.
One factor that was an important determinant of garden placement was available space. We looked at the prevalence of gardens along a gradient of impervious surface across garden sites and derived a model for the percent chance of finding a garden with increasing impervious surface. Community gardens were more likely to be located in places with low levels of impervious surface (Figure 2).
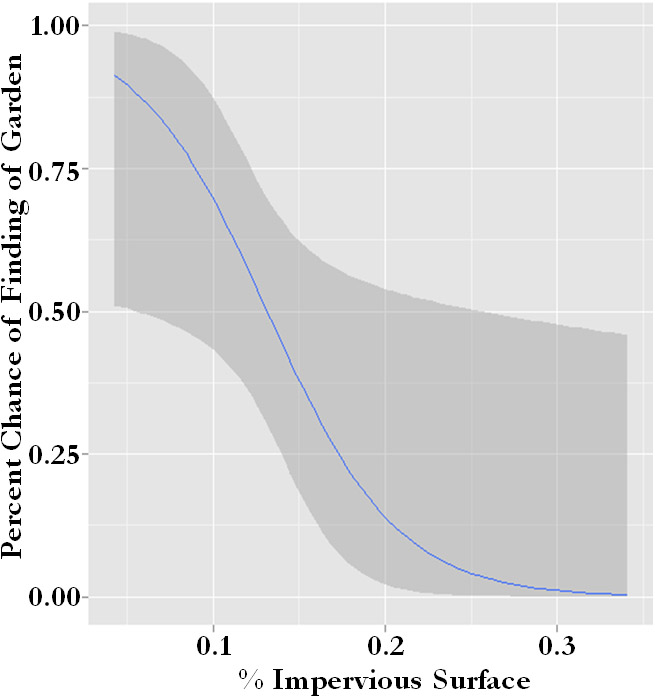
Socio-economic Demographics and Garden Quality
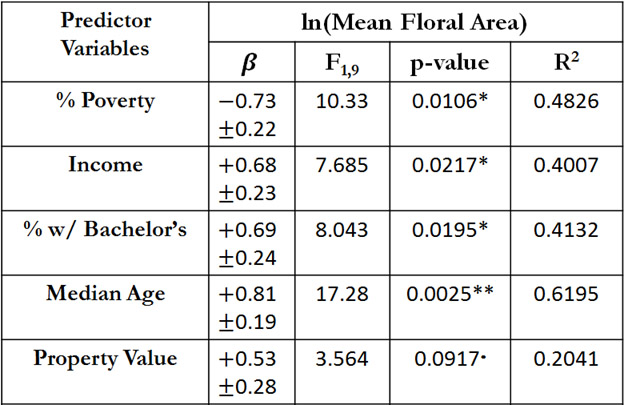
Across the 11 sample sites, 2086 floral abundance measurements were taken of 316 flowering plant species. Results of linear models between mean floral area and socio-economic variables are shown in Table 1. Variables associated with wealth (median income, percent with bachelor’s degree, and median age), had a significant positive linear relationship with general floral resource availability in community gardens. Conversely, percent of residents below the poverty line surrounding gardens had a significant negative correlation with floral area. In general, Ann Arbor/Ypsilanti gardens in more affluent areas tended to have greater overall floral resource availability than those in lower-income communities. In contrast there was a decrease in floral resource abundance associated with poverty. This qualitative result was mirrored in the results of the PLS regression (see Appendix 1.2).
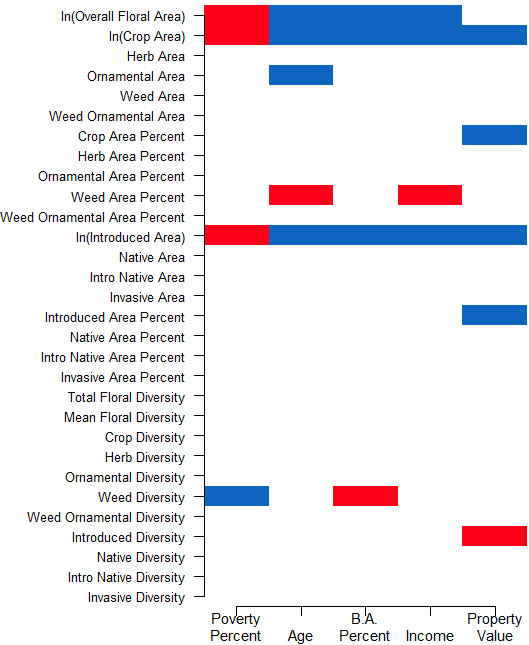
We hypothesized that this increase in floral area associated with wealth did not constitute a proportional increase in all types of flowers. To investigate how floral composition changed with the increase in area, flower data was broken down into further descriptive categories and regressed against the same socio-economic variables as well as general floral area. Significant relationships were found among socio-economic variables and various garden flower composition variables (see Figure 3). Some of the strongest and most consistent relationships involved mean crop area, mean introduced species area, and mean weed percent, and thus these variables will be the primary focus of our analysis.
Significant relationships were found between mean crop area in gardens and all socio-economic variables of interest in surrounding neighborhoods. As with mean floral area, wealth-associated variables were positively correlated with crop area, and poverty was negatively correlated. The same trends held true for mean introduced species floral area: introduced flowers increased with wealth and decreased with poverty. In contrast, the percentage of floral area occupied by weeds had significant negative correlations with income and a positive trend associated with poverty (p=0.052) (see Table 2). It is important to note that we do not see significant changes in absolute weed area with wealth or poverty, but rather the amount of weeds relative to other types of flowers (crops and introduced species) is changing. These relationships were also corroborated in the factors derived from the PLS regression.
Garden Quality and Pollinator Communities

We collected 1,706 individual bee specimens from 112 species. The most abundant species were the bronze sweat bee (Halictus confusus) with 132 individuals, the common eastern bumble bee (Bombus impatiens) with 124 individuals, and a metallic green sweat bee species (Agapostemon virescens) with 116 individuals.
General floral area did not significantly correlate with the number of bees sampled, failing to support the hypothesis that simple increases in general floral resources results in greater bee abundance. In fact, the relationship between floral area and bee abundance trended negative (F1,9=4.76, p=0.057, adjusted R2=0.270), the opposite of what is commonly assumed. This trend might be driven by the significant negative interaction between introduced species floral area and bee abundance (recall that the increase in general floral area was partially comprised of introduced species). On the other hand, there was a nearly significant positive trend (F1,9=5.001 p=0.052, adjusted R2=0.29) between percent weeds and bee abundance; number of bees tended to increase as a greater proportion of garden floral area was occupied by weeds. Finally, there was no significant relationship between crop area and bee abundance.

Discussion & Conclusions
In summary, the analysis of how socio-economic demographics correspond to community garden locations showed significant differences in Ann Arbor between median age and poverty rates in areas surrounding gardens versus non-garden areas. Such differences were not consistent in Ypsilanti, where gardens were more evenly dispersed across neighborhoods. Impervious surface was a better overall predictor of the presence or absence of gardens across both cities. On the other hand, socio-economic factors were closely correlated with garden floral quality and composition. Furthermore, while urban community gardens can be successful in their ability to support pollinator communities, intuitive garden traits within local control might not explain differences in this ability between gardens. In Ann Arbor and Ypsilanti community gardens, we find wealth is correlated with high floral areas, but that does not necessarily translate to more abundant bee communities. The statistical relationships leading to these conclusions are summarized in Figure 5.
In general, the socio-economic makeup of neighborhoods surrounding community gardens tended to reflect the socio-economic makeup of their respective cities. In Ypsilanti, there was no significant difference for any socio-economic variables. In Ann Arbor there was no difference for income, education, or property value, while there were significant differences for poverty and age. The city’s much larger student population might explain these differences in Ann Arbor. Students who are not generally present during the peak growing months tend to live in the denser, centrally located neighborhoods. Ann Arbor residents participating in community gardening are likely to be older, permanent inhabitants living near lower-density residential neighborhoods that contain more open space for gardens. The large student population would thus decrease the median age in areas without gardens relative to those areas where gardens are located. Similarly, the presence of off-campus college students has been shown to skew census poverty rates upward (Bishaw 2013). Thus, we would expect non-garden areas occupied by students to have higher poverty rates than garden areas.
More than socio-economic factors, percentage of area covered by impervious surface seems to be an important predictor of where community gardens are located in a city. This makes intuitive sense—gardens are more likely to get established where there is more available space. The land use types in which gardens tended to occur further support this understanding qualitatively: 77 percent of gardens were on government/institutional land or in public parks/recreational space, while the remaining 23 percent were on commercial or residential land. Beyond this general trend, the maps in Figure 1 illustrate gardens in Ypsilanti were more centrally clustered than in Ann Arbor. The heterogeneity of spatial and demographic characteristics across even the two geographically proximate cities in this work highlights the need for similar studies to be conducted at fine scales across a diversity of locations.
These findings also highlight the importance of land use patterns, city planning, and policy for urban agriculture. The gardens in Ann Arbor and Ypsilanti spanned diverse communities, demonstrating that gardens are established and persist regardless of a community’s characteristics. However, the findings indicate that high presence of impervious surface and lack of available open land may be a difficult barrier to overcome in developing gardens, no matter how strong the environmental, ecological, or community-oriented incentives to do so may be. Vacant land may be unavailable or the cost of land may be insurmountable for an individual or community motivated to develop a community garden. Therefore, strengthening or expanding urban agriculture will require the attention of city planners and policy makers. Urban agriculture-friendly zoning or land use designations, along with initiatives such as providing gardens with city water access or allowing communities to develop gardens in public parks, are some ways in which municipal stakeholders can, and may need to, support community gardens.
Beyond garden location, characteristics of community garden floral quality are significantly correlated with socio-economic variables, indicating that even factors ostensibly within the purview of local- or individual-level decision-making might be influenced by broader demographic trends. Our analysis found that variables associated with wealth, such as low poverty and high income, educational attainment, and age tended to positively correlate with floral area in Ann Arbor and Ypsilanti community gardens. This increase in general floral area was primarily driven by increases in crops and introduced plant species.
Furthermore, there was a negative association between income and percentage of floral area occupied by weeds. Although we do not have qualitative data such as gardener interviews to suggest a causal mechanism for these relationships, we can propose potential explanatory hypotheses. Gardens in wealthier areas, with higher incomes and lower poverty levels, plausibly attract wealthier gardeners who are better resourced and able to manage garden plots more intensively. This could explain the significant increases in crop area (i.e. wealthier gardeners purchase and plant more crops) and corresponding increase in general floral area, as well as the decrease in percent weeds (i.e. wealthier gardeners have more resources, time, and/or concern for weed management). A similar “luxury effect” has been found in other research on vegetation in urban areas, such as a 2003 study which demonstrated socio-economic drivers of plant diversity around Phoenix, AZ (Hope et al. 2003).
One might presume that these characteristics make gardens in wealthier areas “higher quality.” In particular, the significantly higher floral area in affluent gardens seems likely to be more attractive and beneficial to wild bees. Yet our findings do not support this assumption. In fact, increased floral area had a nearly significant negative trend with bee abundance in the 11 community gardens studied. Counter to what one might expect, we found that fewer bees were present as gardens had more flowers.
Parsing general floral area into particular categories might help explain this counterintuitive result. Specifically, increased floral abundance is driven primarily by addition of crops and introduced plant species, and the latter is also negatively correlated with bee abundance. The one floral variable that had a positive trend on bee abundance was the proportion of the floral area occupied by weed plants (“percent weeds”). The value of weeds (non-crop flowers) to pollinators has been well documented, such as in a recent FAO review of the ecological interactions between crops, weeds, and pollinators in agricultural systems (Altieri et al. 2015). Bees tend to be attracted to particular flower types based on nutrient quality, such as pollen protein content (Hanley et al. 2008), and non-crop flowers provide pollinators with diet diversity necessary for healthy development (Alaux et al. 2010; Schmitt et al. 1995). Furthermore, various studies have demonstrated that floral morphology, color, and scent are all important components to bee foraging decisions (Harder 1985; Kunze and Gumbert 2001). It is therefore plausible that increased proportion of crop and/or introduced flowers relative to weeds in gardens “dampens the signal” of weed flowers that are attractive to wild bees. Ultimately these results suggest that while urban community gardens are capable of supporting bee communities, moving beyond a paradigm of simple floral additions is key to sustainability. The composition of the floral resources in gardens matters, and it could be valuable for urban gardeners to incorporate more non-crop, wild flowers along with crops in order to fully support bee health.
Our study design necessarily entails limitations that should be accounted for when extrapolating results. First, using census data conducted at such a broad scale is not a perfect on-the-ground measurement of the socio-economic reality of the communities surrounding urban gardens or the wealth status of gardeners themselves. Flower and bee sampling only took place over one growing season, and thus cannot account for longer-term temporal variation in community abundance and composition. Furthermore, our analyses did not account for other garden qualities besides floral resources such as nesting habitat availability. Finally, definitive causal relationships can only be inferred due to both the observational nature of our study and the small sample size in a specific local context. Yet our results suggest future research directions into similar trends in other urban contexts as well as the mechanistic interactions among socio-economic drivers, garden location and quality, and pollinator communities. Perhaps most importantly, the interdisciplinary methodology employed here exemplifies a novel and potentially useful tool for addressing complex questions associated with social and ecological sustainability in cities. If urban gardens are anticipated to have wide-reaching effects that cut across socio-cultural, economic, public health, and environmental dimensions, research methods to study these effects must be similarly broad and diverse. Our study constitutes an illustrative example of one such approach. Incorporating similar methods into future research questions could deepen understanding of the broader relationships between socio-economic characteristics, land use, and the prevalence of community gardens, and identify the best associated policy recommendations that would foster the expansion of urban agriculture and improve conditions for native bee populations.
Acknowledgements
We would like to acknowledge the gardeners in Project Grow and Growing Hope who allowed us access into their plots to complete the biodiversity surveys. Additionally, we thank Brad Vogelsmeier for his work on completing the GIS mapping for the garden sites. We would also like to thank Maria Carolina Simao, Gordon Fitch, and Chatura Vaidya for their work in completing the biodiversity survey and overall flower metadata list. Finally, we would like to thank Professor Robert Goodspeed for allowing author Paul Glaum the chance to introduce this project to his GIS course.
References
- Alaux, C., F. Ducloz, D. Crauser, and Y. Le Conte. “Diet Effects on Honeybee Immunocompetence.” Biology Letters 6, no. 4 (January 20, 2010): 562–565. doi:10.1098/rsbl.2009.0986.
- Altieri, Miguel A., Clara I. Nichols, Mark Gillespie, Ben Waterhouse, Steve Wratten, Gualbert Gbèhounou, and Barbara Gemmill-Herren. “Crops, weeds and pollinators: Understanding ecological interaction for better management.” Food and Agriculture Organization of the United Nations, (2015). http://www.fao.org/3/a-i3821e.pdf.
- Banaszak, Józef, Weronika Banaszak-Cibicka, and Piotr Szefer. “Guidelines on Sampling Intensity of Bees (Hymenoptera: Apoidea: Apiformes).” Journal of Insect Conservation 18, no. 4 (July 26, 2014): 651–656. doi:10.1007/s10841-014-9671-3.
- Bishaw, Alemayehu. “Examining the Effect of Off-Campus College Students on Poverty Rates.” U. S. Census Bureau, Social, Economic & Housing Statistics Division, Poverty
- Statistics Branch, (May 1, 2013). http://www.census.gov/content/dam/Census/library/working-papers/2013/acs/2013_Bishaw_01.pdf
- Blacquiere, T., Smagghe, G., Van Gestel, C. A., & Mommaerts, V. (2012). Neonicotinoids in bees: a review on concentrations, side-effects and risk assessment. Ecotoxicology, 21(4), 973-992. doi: 10.1007/s10646-012-0863-x
- Chatterjee, S.; Hadi, A. S.; Price, B. (2000). Regression Analysis by Example (Third ed.). John Wiley and Sons. ISBN 0-471-31946-5
- Clifford H. Wagner (February 1982). “Simpson's Paradox in Real Life.” The American Statistician. 36 (1): 46–48. doi:10.2307/2684093.
- Fortel, Laura, Mickaël Henry, Laurent Guilbaud, Anne Laure Guirao, Michael Kuhlmann, Hugues Mouret, Orianne Rollin, and Bernard E. Vaissière. “Decreasing Abundance, Increasing Diversity and Changing Structure of the Wild Bee Community (Hymenoptera: Anthophila) Along an Urbanization Gradient.” Edited by M. Alex Smith. PLoS ONE 9, no. 8 (August 13, 2014): e104679. doi:10.1371/journal.pone.0104679.
- Gallai, Nicola, Jean-Michel Salles, Josef Settele, and Bernard E. Vaissière. “Economic Valuation of the Vulnerability of World Agriculture Confronted with Pollinator Decline.” Ecological Economics 68, no. 3 (January 2009): 810–821. doi:10.1016/j.ecolecon.2008.06.014.
- Galluzzi, Gea, Pablo Eyzaguirre, and Valeria Negri. “Home Gardens: Neglected Hotspots of Agro-Biodiversity and Cultural Diversity.” Biodiversity and Conservation 19, no. 13 (September 17, 2010): 3635–3654. doi:10.1007/s10531-010-9919-5.
- Garibaldi, L. A., I. Steffan-Dewenter, R. Winfree, M. A. Aizen, R. Bommarco, S. A. Cunningham, C. Kremen, et al. “Wild Pollinators Enhance Fruit Set of Crops Regardless of Honey Bee Abundance.” Science 339, no. 6127 (February 28, 2013): 1608–1611. doi:10.1126/science.1230200.
- Garibaldi, L. A., M. A. Aizen, A. M. Klein, S. A. Cunningham, and L. D. Harder. “Global Growth and Stability of Agricultural Yield Decrease with Pollinator Dependence.” Proceedings of the National Academy of Sciences 108, no. 14 (March 21, 2011): 5909–5914. doi:10.1073/pnas.1012431108.
- Goulson, D., E. Nicholls, C. Botias, and E. L. Rotheray. “Bee Declines Driven by Combined Stress from Parasites, Pesticides, and Lack of Flowers.” Science 347, no. 6229 (February 26, 2015): 1255957–1255957. doi:10.1126/science.1255957.
- Grove, Jeremiah M., Arlington R. Troy, John PM O’Neil-Dunne, W. R. Burch, M. L. Cadenasso, and S. T. A. Pickett. “Characterization of households and its implications for the vegetation of urban ecosystems.” Ecosystems 9, no. 4 (2006): 578-597. doi:10.1007/s10021-006-0116-z.
- Guitart, Daniela, Catherine Pickering, and Jason Byrne. “Past Results and Future Directions in Urban Community Gardens Research.” Urban Forestry & Urban Greening 11, no. 4 (January 2012): 364–373. doi:10.1016/j.ufug.2012.06.007.
- Haenlein, Michael, and Andreas M. Kaplan. “A beginner's guide to partial least squares analysis.” Understanding Statistics 3.4 (2004): 283-297.
- Hanley, M. E., M. Franco, S. Pichon, B. Darvill, and D. Goulson. “Breeding System, Pollinator Choice and Variation in Pollen Quality in British Herbaceous Plants.” Functional Ecology 22, no. 4 (August 2008): 592–598. doi:10.1111/j.1365-2435.2008.01415.x.
- Harder, Lawrence D. “Morphology as a Predictor of Flower Choice by Bumble Bees.” Ecology 66, no. 1 (February 1985): 198–210. doi:10.2307/1941320.
- Hernandez, Jennifer L., Gordon W. Frankie, and Robbin W. Thorp. “Ecology of urban bees: a review of current knowledge and directions for future study.” Cities and the Environment (CATE) 2, no. 1 (2009): 3. http://digitalcommons.lmu.edu/cgi/viewcontent.cgi?article=1036&context=cate.
- Hope, Diane, Corinna Gries, Weixing Zhu, William F. Fagan, Charles L. Redman, Nancy B. Grimm, Amy L. Nelson, Chris Martin, and Ann Kinzig. “Socio-economics Drive Urban Plant Diversity.” Urban Ecology (2003): 339–347. doi:10.1007/978-0-387-73412-5_21.
- Inouye, David, Samuel Droege, and Jonathan Mawdsley. “Words alone will not protect pollinators.” Science 355, no. 6323 (2017): 357-357. doi:10.1126/science.aam6132.
- IPBES, The Assessment Report of the Intergovernmental Science-Policy Platform on Biodiversity and Ecosystem Services on Pollinators, Pollination, and Food Production (IPBES, Bonn, Germany, 2016). http://www.ipbes.net/sites/default/files/downloads/pdf/spm_deliverable_3a_pollination_20170222.pdf.
- Janhäll, Sara. “Review on Urban Vegetation and Particle Air Pollution—Deposition and Dispersion.” Atmospheric Environment 105 (March 2015): 130–137. doi:10.1016/j.atmosenv.2015.01.052.
- Kinzig, Ann, Paige Warren, Chris Martin, Diane Hope, and Madhusudan Katti. “The effects of human socio-economic status and cultural characteristics on urban patterns of biodiversity.” Ecology and Society 10, no. 1 (2005). doi:10.5751/ES-01264-100123.
- Kulak, Michal, Anil Graves, and Julia Chatterton. “Reducing Greenhouse Gas Emissions with Urban Agriculture: A Life Cycle Assessment Perspective.” Landscape and Urban Planning 111 (March 2013): 68–78. doi:10.1016/j.landurbplan.2012.11.007.
- Kunze, Jan, and Andreas Gumbert. “The combined effect of color and odor on flower choice behavior of bumble bees in flower mimicry systems.” Behavioral Ecology 12, no. 4 (2001): 447-456. doi:10.1093/beheco/12.4.447.
- Loss, Scott R., Marilyn O. Ruiz, and Jeffrey D. Brawn. “Relationships between avian diversity, neighborhood age, income, and environmental characteristics of an urban landscape.” Biological Conservation 142, no. 11 (2009): 2578-2585. doi:10.1016/j.biocon.2009.06.004
- Matteson, Kevin C., and Gail A. Langellotto. “Small Scale Additions of Native Plants Fail to Increase Beneficial Insect Richness in Urban Gardens.” Insect Conservation and Diversity 4, no. 2 (April 7, 2011): 89–98. doi:10.1111/j.1752-4598.2010.00103.x.
- Matteson, Kevin C., John S. Ascher, and Gail A. Langellotto. “Bee richness and abundance in New York City urban gardens.” Annals of the Entomological Society of America 101, no. 1 (2008): 140-150. doi:10.1603/0013-8746(2008)101[140:BRAAIN]2.0.CO;2.
- Mevik, Björn-Helge, and Ron Wehrens. “The PLS package: principal component and partial least squares regression in R.” Journal of Statistical software 18.2 (2007): 1-24.
- Pardee, Gabriella L., and Stacy M. Philpott. “Native Plants Are the Bee’s Knees: Local and Landscape Predictors of Bee Richness and Abundance in Backyard Gardens.” Urban Ecosystems 17, no. 3 (January 23, 2014): 641–659. doi:10.1007/s11252-014-0349-0.
- Potter, Andrew, and Gretchen LeBuhn. “Pollination Service to Urban Agriculture in San Francisco, CA.” Urban Ecosystems 18, no. 3 (January 14, 2015): 885–893. doi:10.1007/s11252-015-0435-y.
- Potts, Simon G., Jacobus C. Biesmeijer, Claire Kremen, Peter Neumann, Oliver Schweiger, and William E. Kunin. “Global Pollinator Declines: Trends, Impacts and Drivers.” Trends in Ecology & Evolution 25, no. 6 (June 2010): 345–353. doi:10.1016/j.tree.2010.01.007.
- National Gardening Association. “Garden to Table: A 5 Year Look at Food Gardening in America” National Gardening Association, (2014). https://garden.org/special/pdf/2014-NGA-Garden-to-Table.pdf.
- O’Brien, R. M. (2007). “A Caution Regarding Rules of Thumb for Variance Inflation Factors”. Quality & Quantity. 41 (5): 673. doi:10.1007/s11135-006-9018-6.
- Santo, R., A. Palmer, and B. Kim. “Vacant lots to vibrant plots: a review of the benefits and limitations of urban agriculture.” John Hopkins Center for a Livable Future, May (2016). http://www.jhsph.edu/research/centers-and-institutes/johns-hopkins-center-for-a-livable-future/_pdf/research/clf_reports/urban-ag-literature-review.pdf.
- Schmidt, L. S., J. O. Schmidt, H. Rao, W. Wang, and L. Xu. “Feeding Preference and Survival of Young Worker Honey Bees (Hymenoptera: Apidae) Fed Rape, Sesame, and Sunflower Pollen.” Journal of Economic Entomology 88, no. 6 (December 1, 1995): 1591–1595. doi:10.1093/jee/88.6.1591.
- Smith, Richard M., Philip H. Warren, Ken Thompson, and Kevin J. Gaston. “Urban domestic gardens (VI): environmental correlates of invertebrate species richness.” In human exploitation and biodiversity conservation, pp. 75-98. Springer Netherlands, 2005. doi:10.1007/s10531-004-5014-0.
- Woodcock, Ben A., Nicholas JB Isaac, James M. Bullock, David B. Roy, David G. Garthwaite, Andrew Crowe, and Richard F. Pywell. “Impacts of neonicotinoid use on long-term population changes in wild bees in England.” Nature Communications 7 (2016): 12459. doi:10.1038/ncomms12459.
Appendix 1
Appendix 1.1 - Data Transformations
Given the use of linear models in this analysis, variables were tested for normality using the Shapiro Wilks test. Upon passing the Shapiro Wilks test, skewed variables with non-normal distributions were log transformed. All transformed variables were reexamined using normal Q-Q plots and the Shapiro Wilks test to verify better fits to normality.
Appendix 1.2 Addressing Multicollinearity
Socio-economic variables are often related to each other. This is intuitive given that an area’s general income levels are inversely proportional to poverty rates but directly proportional to property values. When such socio-economic variables are used as predictors in multivariate regression analysis, this can lead to issues of multicollinearity. In general, this means that the predictor variables (the socio-economic data in our case) are all linearly correlated with each other. An example of what this multicollinearity looks like is displayed in Appendix Figure 1 with our data; notice the consistent linear relations between all the predictor variables. Multicollinearity must be recognized because it obfuscates the precision in multivariate models using ordinary least squares regression to estimate coefficients.
When multicollinear variables are used as predictors in ordinary multivariate regressions, it can be a considerable problem because multicollinearity can make coefficient estimates imprecise. Additionally, multicollinearity increases the standard errors of the estimated coefficients (Chatterjee et al. 2000). Increased standard errors, in turn, mean that coefficients for some predictor variables may be found not to be significantly different from zero. In other words, by over-inflating the standard errors, multicollinearity makes some variables statistically insignificant when they should be significant. Without multicollinearity (and thus, with lower standard errors), those coefficients may be significant. Therefore, before proceeding, we tested for multicollinearity in our models.

To test the degree of multicollinearity in our data, we measured variance-inflated factors (VIF). VIF values larger than five indicate multicollinearity problems that must be addressed (O’Brien 2007). Our data set produced VIF values as high as 25 without interactions between predictor variables and as high as 160 when interactions were directly accounted for in models (e.g. Poverty*Income in linear regressions). We can conclude, then, that our data exhibits a high degree of multicollinearity, and ordinary multivariate statistics/regressions are not appropriate for analysis in this case.
One method of analysis we used to avoid the issues of multicollinearity was partial least squares regression (PLS regression). PLS regressions create estimates using partial least squares rather than ordinary least squares (as the name implies). This helps avoid the issues of collinearity between predictors. PLS regression is often used when predictor variables are highly collinear (Mevik and Wehrens 2007). PLS regressions work in a similar manner to principal component analysis (PCA) in that it finds independent linear combinations of the predictor variables (our socio-economic data) that best predict the dependent variable (e.g. floral data). These combinations are called factors or components. The elements of these factors/components are regression coefficients for each predictor variable. We chose the appropriate number of components per regression using cross-validation. For more in-depth explanations on PLS, see Haenlein (2004). For the purposes of this work, PLS was used to find the appropriate factors and their regression coefficients, which we could then compare to the more common beta coefficients obtained from our single-variate models. This acted as a degree of authentication of the relationships found between socio-economic variables and dependent variables.
For example, when using PLS we can run the following model in R:
pls( ln(Mean Floral Area) ~ (Poverty) + (Income) + (BA Percentage) + (Median Age)+(Property Value) )
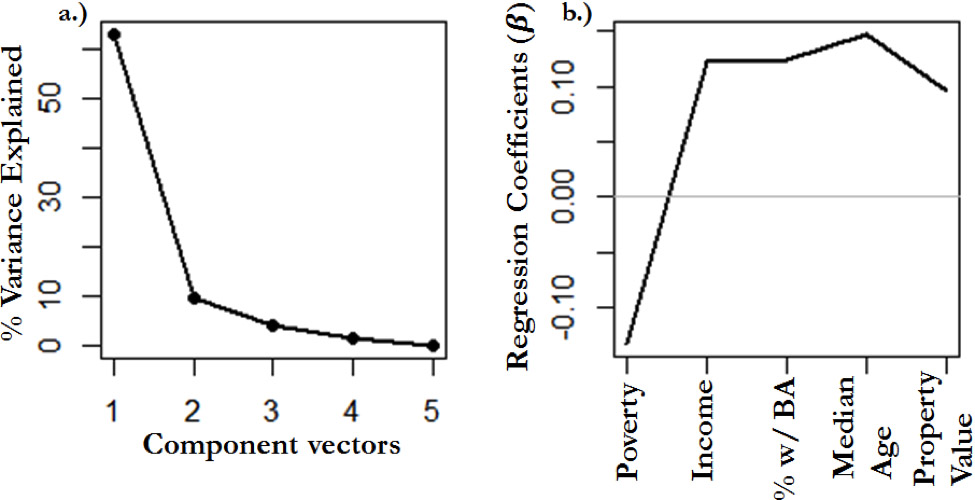
This produces five component vectors/factors, each one of which explains a certain amount of the variance. As is visible in Appendix Figure 2a, component 1 explains the most variance by far. The other component vectors add little to explanative power of the regression. The coefficients of each predictor variable are shown in Appendix Figure 2b. Poverty shows a negative relationship while all other variables associated with wealth show a positive relationship. These coefficients correspond in their +/- sign from those detailed in Table 1. Similar corresponding PLS component vectors can be produced for all results detailed in Table 2.